An underwater target recognition method based on the fusion of GRU and one-dimensional CNN neural network
An underwater target, neural network technology, applied in neural learning methods, biological neural network models, neural architectures, etc., can solve the problem of inability to extract temporal features, and achieve the effect of improving recognition accuracy
- Summary
- Abstract
- Description
- Claims
- Application Information
AI Technical Summary
Problems solved by technology
Method used
Image
Examples
Embodiment Construction
[0048] Below in conjunction with accompanying drawing, the implementation of the present invention is described in detail, and provide concrete mode of operation and implementation steps:
[0049] A kind of underwater target recognition method based on the fusion of GRU and one-dimensional CNN neural network mainly includes the following steps:
[0050] 1. Collect the underwater radiation noise data and auxiliary information of ships measured in real ocean experiments, and train the underwater target recognition neural network based on the fusion of GRU and one-dimensional CNN.
[0051] The training steps of the underwater target recognition neural network based on the fusion of GRU and one-dimensional CNN are as follows:
[0052] Step 1: From the ocean test, the noise data of the underwater radiation of the ship is measured by using the hydrophone fixed in advance; at the same time, the distance between the hydrophone and the target ship, the actual depth of the hydrophone, a...
PUM
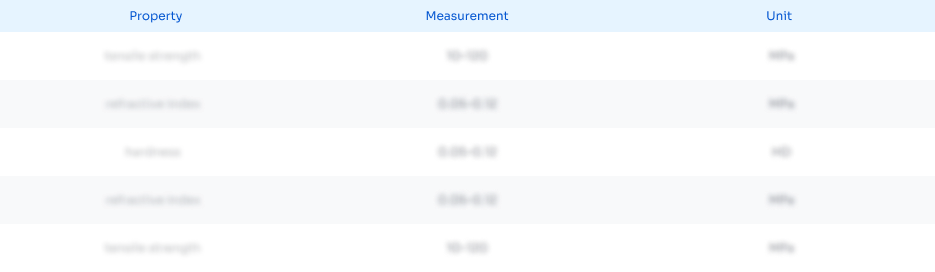
Abstract
Description
Claims
Application Information
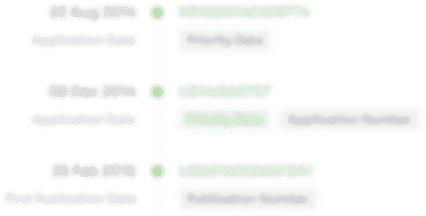
- Generate Ideas
- Intellectual Property
- Life Sciences
- Materials
- Tech Scout
- Unparalleled Data Quality
- Higher Quality Content
- 60% Fewer Hallucinations
Browse by: Latest US Patents, China's latest patents, Technical Efficacy Thesaurus, Application Domain, Technology Topic, Popular Technical Reports.
© 2025 PatSnap. All rights reserved.Legal|Privacy policy|Modern Slavery Act Transparency Statement|Sitemap|About US| Contact US: help@patsnap.com