Transformer operation state prediction method based on long short term memory network and transformer operation state prediction system based on long short term memory network
A long- and short-term memory and operating state technology, applied in neural learning methods, biological neural network models, instruments, etc., can solve problems such as threats to normal production and life, damage to transformers, etc.
- Summary
- Abstract
- Description
- Claims
- Application Information
AI Technical Summary
Problems solved by technology
Method used
Image
Examples
example 1
[0100] In order to increase the learning speed and effect, and reduce the risk of the network falling into a local minimum during learning, the weight matrix in LSTM is first randomly initialized by obeying a Gaussian distribution with a mean of 0 and a variance of 1, and then using singular value decomposition to obtain an orthogonal base matrix as an initialization value [22] . The LSTM bias item and the output layer bias item are initialized to 0, and the output layer weight matrix is multiplied by 0.01 by a random number that obeys a Gaussian distribution with a mean of 0 and a variance of 1. The prediction model consists of one layer of LSTM network and Softmax network layer. The size of the input layer is 100, and the number of neurons in the hidden layer of LSTM is 128. To prevent overfitting, the signal loss rate is set to 0.2, and the size of the output layer is 4.
[0101] Taking a 500kV #2 main transformer as an example, the basic situation of the transformer is...
example 2
[0107] Taking a 220kV #1 main transformer as an example, the transformer was shipped in April 2000 and put into operation on June 23, 2000. After it was put into operation, the operation of the main transformer was basically good, and the overall load rate was relatively high. Periodic chromatographic testing found that the total hydrocarbon value in the main transformer oil increased significantly after the main transformer reached its peak in 2010 and passed the summer. The value of total hydrocarbons increased slowly year by year, but did not exceed the value for attention, and the data of other characteristic gases except total hydrocarbons were normal. Table 2 shows some oil chromatographic online monitoring data from June to July 2013.
[0108] Table 2. Online monitoring data of a 220kV #1 main transformer oil chromatography (unit: uL / L)
[0109] time
H 2
CH 4
C 2 h 4
C 2 h 6
C 2 h 2
total hydrocarbon
CO
CO 2
...
PUM
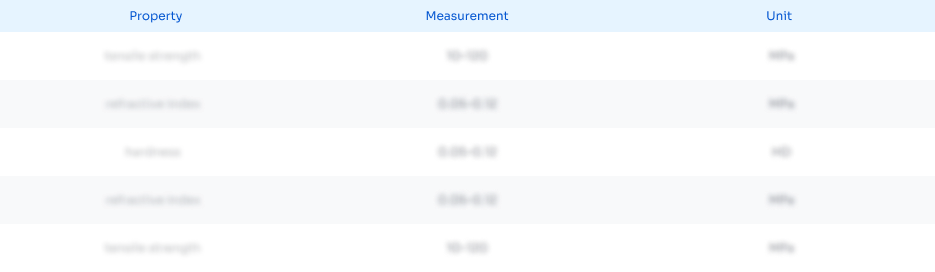
Abstract
Description
Claims
Application Information
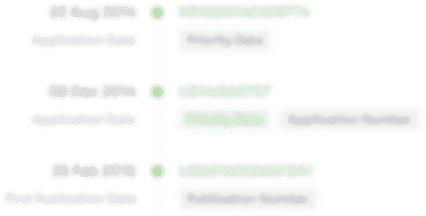
- R&D Engineer
- R&D Manager
- IP Professional
- Industry Leading Data Capabilities
- Powerful AI technology
- Patent DNA Extraction
Browse by: Latest US Patents, China's latest patents, Technical Efficacy Thesaurus, Application Domain, Technology Topic, Popular Technical Reports.
© 2024 PatSnap. All rights reserved.Legal|Privacy policy|Modern Slavery Act Transparency Statement|Sitemap|About US| Contact US: help@patsnap.com