Multi-wave seismic oil and gas reservoir prediction method under unsupervised and supervised learning
An unsupervised learning and supervised learning technology, applied in the field of multi-wave seismic oil and gas reservoir prediction, to reduce redundancy and improve efficiency
- Summary
- Abstract
- Description
- Claims
- Application Information
AI Technical Summary
Problems solved by technology
Method used
Image
Examples
Embodiment Construction
[0031] Glossary:
[0032] Unsupervised learning (unsupervised learning): There is no training data sample in advance, and the data needs to be modeled directly. That is, at this time, the data has no category information, and no target value will be given. In unsupervised learning, the process of dividing a data set into multiple classes consisting of similar objects is called cluster analysis.
[0033] Supervised learning: Train with existing training samples (that is, known data and their corresponding outputs) to obtain an optimal model, and then use this model to map all new data samples to corresponding output results , make simple judgments on the output results to achieve the purpose of classification, then this optimal model also has the ability to classify unknown data. Unsupervised learning can reduce the dimensionality of data features, that is, unsupervised results can greatly improve the effect of the next step of processing. For example, through cluster analys...
PUM
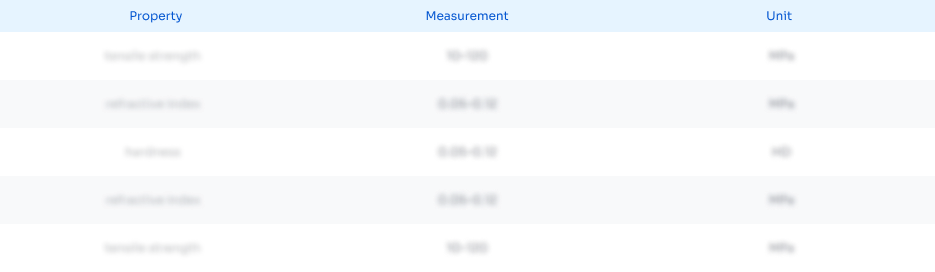
Abstract
Description
Claims
Application Information
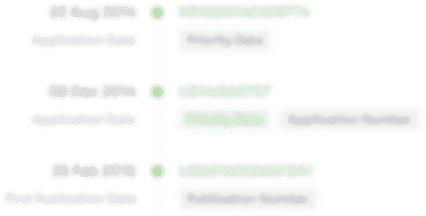
- R&D Engineer
- R&D Manager
- IP Professional
- Industry Leading Data Capabilities
- Powerful AI technology
- Patent DNA Extraction
Browse by: Latest US Patents, China's latest patents, Technical Efficacy Thesaurus, Application Domain, Technology Topic.
© 2024 PatSnap. All rights reserved.Legal|Privacy policy|Modern Slavery Act Transparency Statement|Sitemap