Entity relation extraction method
An entity relationship and relationship technology, applied in the field of information processing, can solve problems such as high labor costs and inability to deal with Internet volume entities and relationship information.
- Summary
- Abstract
- Description
- Claims
- Application Information
AI Technical Summary
Problems solved by technology
Method used
Image
Examples
Embodiment 1
[0073] Please refer to figure 1 , a flowchart of an entity relationship extraction method. The entity relationship extraction methods disclosed in this application include:
[0074] S301. Input the preprocessing information into the word sequence neural network to extract the first type of extraction relationship. The preprocessing information includes several sentences, and the sentences can be obtained directly from text information or voice information. Extracting relationships includes outputting various relationships represented by preprocessing information and the probabilities of the respective relationships.
[0075] Query the entity referred to by each word in the preprocessing information based on the knowledge graph, and convert the preprocessing information into an entity sequence according to the order of the entities in the preprocessing information;
[0076] Perform word segmentation on the preprocessing information to obtain several words; convert the prepro...
Embodiment 2
[0100] Please refer to figure 2 with image 3 , figure 2 It is a flowchart of an entity relationship extraction method, image 3 It is a schematic diagram of the structure of an entity relationship extraction method. The entity relationship extraction method in this embodiment includes:
[0101] S401. Transform the preprocessed information into an entity sequence, specifically including:
[0102] Through n-gram (referring to n words that appear consecutively) text matching, the sequence of appearance of entities in the preprocessing information is linked to the knowledge graph used, while retaining the link of each entity to refer to the candidate entity. Wherein, entity linking (EntityLinking), or entity linking, refers to linking the name appearing in the preprocessing information to the entity it refers to. In natural language, multiple entities may share the same name, that is, names may be ambiguous. For example, the name "Washington" can refer not only to the fir...
PUM
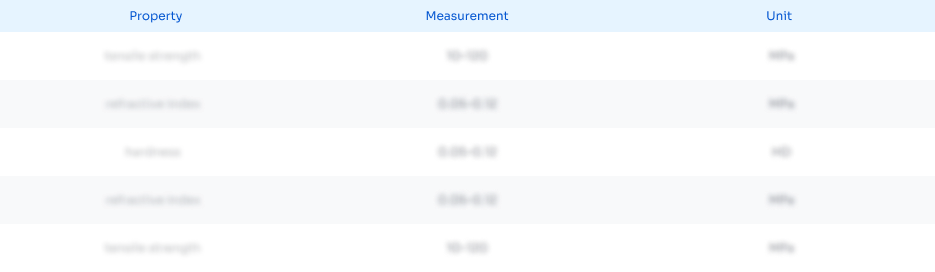
Abstract
Description
Claims
Application Information
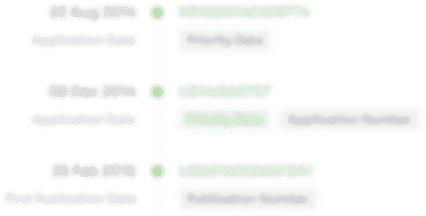
- R&D Engineer
- R&D Manager
- IP Professional
- Industry Leading Data Capabilities
- Powerful AI technology
- Patent DNA Extraction
Browse by: Latest US Patents, China's latest patents, Technical Efficacy Thesaurus, Application Domain, Technology Topic, Popular Technical Reports.
© 2024 PatSnap. All rights reserved.Legal|Privacy policy|Modern Slavery Act Transparency Statement|Sitemap|About US| Contact US: help@patsnap.com