Maximum correlation principal component analysis method based on deep parameter learning
A principal component analysis and depth parameter technology, applied in the field of data processing, can solve problems such as multiple storage spaces and computing costs, hinder analysis of data correlation, and increase the difficulty of data analysis
- Summary
- Abstract
- Description
- Claims
- Application Information
AI Technical Summary
Problems solved by technology
Method used
Image
Examples
Embodiment Construction
[0012] This method of maximum correlation principal component analysis based on deep parameter learning uses deep parameterization to approach unknown but existing nonlinear functions, maps high-dimensional data with nonlinear structures to data of the same dimension with linear structures, and then uses Principal component analysis reduces the dimensionality of the data.
[0013] The present invention parameterizes the transfer operator through learning. The method uses independent mapping chains for each feature, which is similar to the forward propagation structure of the neural network, but the interaction between variables is independent. Therefore, each mapping chain has only one input variable and approximates a non-linear transition operator. This method uses the deep parameterization method to approximate the unknown but existing nonlinear function, maps the high-dimensional data with a nonlinear structure to the same-dimensional data with a linear structure, and then...
PUM
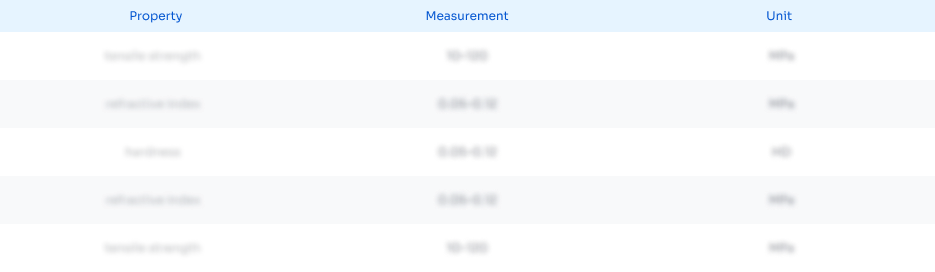
Abstract
Description
Claims
Application Information
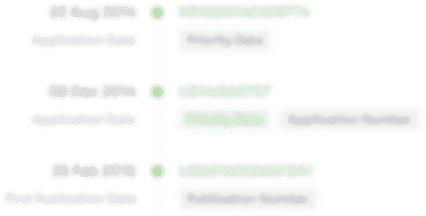
- R&D Engineer
- R&D Manager
- IP Professional
- Industry Leading Data Capabilities
- Powerful AI technology
- Patent DNA Extraction
Browse by: Latest US Patents, China's latest patents, Technical Efficacy Thesaurus, Application Domain, Technology Topic, Popular Technical Reports.
© 2024 PatSnap. All rights reserved.Legal|Privacy policy|Modern Slavery Act Transparency Statement|Sitemap|About US| Contact US: help@patsnap.com