A method and system for dialogue reply generation based on self-comment sequence learning
A technology of sequence and computer system, applied in the field of dialogue reply generation based on self-comment sequence learning, can solve the problems of difficult initialization strategy, mismatch of objective functions, huge word space, etc., to improve the efficiency of text generation and reduce the word search space. Effect
- Summary
- Abstract
- Description
- Claims
- Application Information
AI Technical Summary
Problems solved by technology
Method used
Image
Examples
Embodiment Construction
[0062] The present invention will be further elaborated and illustrated below in conjunction with the accompanying drawings and specific embodiments.
[0063] Such as figure 1 As shown, the present invention divides the encoder-decoder framework into a training module and a testing module, and puts these two modules into the optimization process of the whole model synchronously. Among them, (a) module is a training module, (b) module is a testing module, and the specific steps are as follows:
[0064] (a) Use cross entropy to learn the generation probability between each word in the text generation training process, the basic steps are as follows:
[0065] 1. Initialize the model parameters. For each sentence input, there is a start character , and initialize the hidden state h 0 and unit c 0 as input.
[0066] 2. For each unit, enter the hidden state h of the previous unit i and c i , and the newly entered word w i , after the learning of the recurrent neural ...
PUM
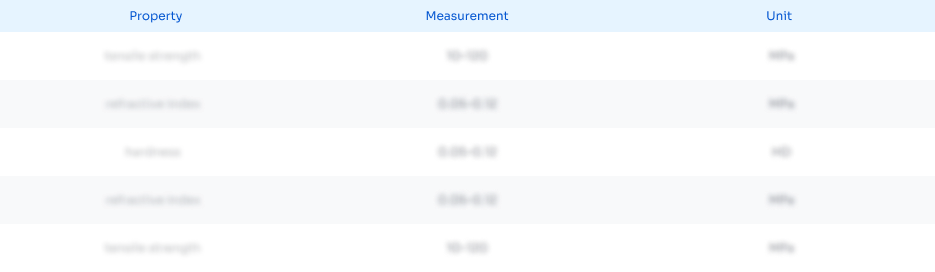
Abstract
Description
Claims
Application Information
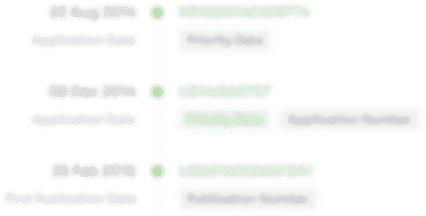
- R&D
- Intellectual Property
- Life Sciences
- Materials
- Tech Scout
- Unparalleled Data Quality
- Higher Quality Content
- 60% Fewer Hallucinations
Browse by: Latest US Patents, China's latest patents, Technical Efficacy Thesaurus, Application Domain, Technology Topic, Popular Technical Reports.
© 2025 PatSnap. All rights reserved.Legal|Privacy policy|Modern Slavery Act Transparency Statement|Sitemap|About US| Contact US: help@patsnap.com