Convolutional neural network filter pruning technology based on similarity learning
A convolutional neural network and filter technology, which is applied in the field of neural networks, can solve problems such as inability to set the filter to zero, loss of precision, and damage to the network structure, and achieve good representation ability and precision, high precision and efficiency.
- Summary
- Abstract
- Description
- Claims
- Application Information
AI Technical Summary
Problems solved by technology
Method used
Image
Examples
Embodiment Construction
[0030] A convolutional neural network filter pruning technique based on similarity learning, such as figure 1 Shown, including:
[0031] S1. Similarity investigation
[0032] Such as figure 2 As shown, the similarity investigation of the present invention is to indirectly estimate the similarity of the filter itself through the similarity of the output of the filter on a given data set. For example, for two filters in a certain layer of the input network, for the same input (such as a picture), these two filters generate two feature matrices; for the same given data set containing multiple pictures, the two Two filters produce two matrix sequences. However, it is actually not feasible to directly use the similarity of the two matrix sequences as the similarity of the two filters. Take the 64 filters of the first layer of ResNet-50 on the ImageNet dataset as an example. If only 1% of the ImageNet training set (12800 pictures) is used as the survey data set, 32-bit floating point ...
PUM
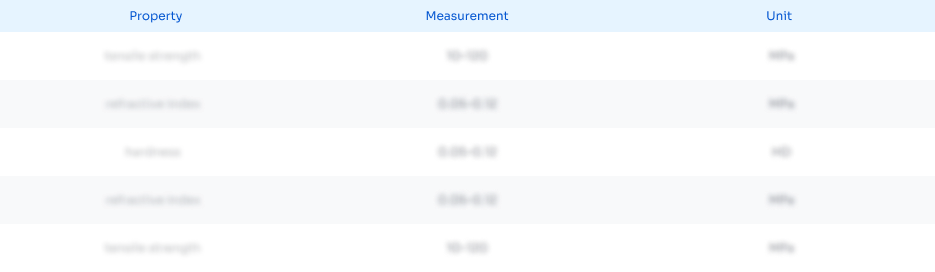
Abstract
Description
Claims
Application Information
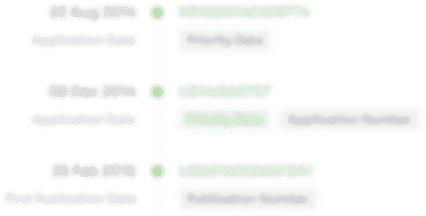
- R&D Engineer
- R&D Manager
- IP Professional
- Industry Leading Data Capabilities
- Powerful AI technology
- Patent DNA Extraction
Browse by: Latest US Patents, China's latest patents, Technical Efficacy Thesaurus, Application Domain, Technology Topic, Popular Technical Reports.
© 2024 PatSnap. All rights reserved.Legal|Privacy policy|Modern Slavery Act Transparency Statement|Sitemap|About US| Contact US: help@patsnap.com