Ship target detection method based on joint training of deep learning features and visual features
A technology of deep learning and target detection, applied in neural learning methods, instruments, biological neural network models, etc., can solve problems such as poor comprehension, different degree of feature retention, and inconsistent ship detection results, achieving high accuracy, fast speed, Highly robust effect
- Summary
- Abstract
- Description
- Claims
- Application Information
AI Technical Summary
Problems solved by technology
Method used
Image
Examples
Embodiment Construction
[0040]The present invention will be further described in connection with the accompanying drawings and examples in conjunction with the accompanying drawings and examples.
[0041]Seefigure 1 The method of the embodiment of the present invention includes the following steps:
[0042]1 sample data collection.
[0043]The data required for the present invention is mainly monitored to monitor video frame data in a coastal region of visible light. For collected video data, each frame image can be obtained by decoding extraction, and the size is 1920 × 1080 pixels. According to the standard of the Pascal Voc, the image containing the vessel target is labeled, the resulting label file is the four vertex coordinates and corresponding images of the minimum surrounding rectangle of the vessel target on each picture, thus constructing a vessel image sample library. .
[0044]2cnn feature extraction.
[0045]The sample obtained by step 1 is unified to 224 × 224 size, then enter into the convolutional neural ...
PUM
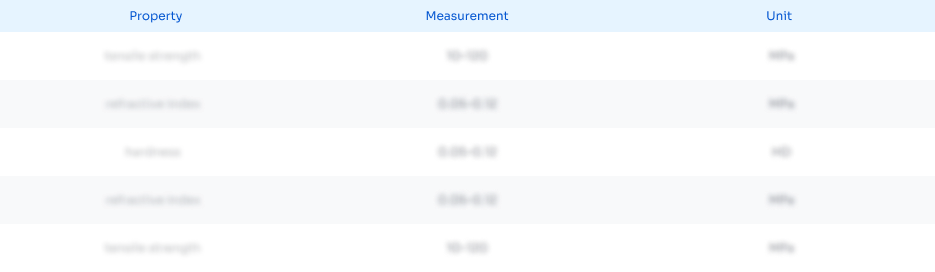
Abstract
Description
Claims
Application Information
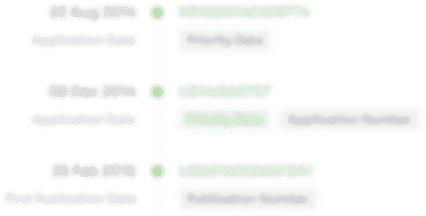
- R&D Engineer
- R&D Manager
- IP Professional
- Industry Leading Data Capabilities
- Powerful AI technology
- Patent DNA Extraction
Browse by: Latest US Patents, China's latest patents, Technical Efficacy Thesaurus, Application Domain, Technology Topic, Popular Technical Reports.
© 2024 PatSnap. All rights reserved.Legal|Privacy policy|Modern Slavery Act Transparency Statement|Sitemap|About US| Contact US: help@patsnap.com