AUV pipeline looping method based on deep reinforcement learning of image features
A technology of reinforcement learning and image features, applied in height or depth control, vehicle position/route/height control, instruments, etc., can solve the difficulty of ensuring the accuracy and reliability of AUV position and pose information, and the accuracy of position and speed information Sensitive, difficult to obtain position and pose information and other issues, to achieve the effect of improving adaptive ability, robust attitude parameter accuracy, and low hardware and software costs
- Summary
- Abstract
- Description
- Claims
- Application Information
AI Technical Summary
Problems solved by technology
Method used
Image
Examples
Embodiment Construction
[0062] The AUV pipeline following method based on deep reinforcement learning of image features of the present invention is carried out sequentially according to the following steps:
[0063] Step 1 Establish two policy models according to the definition of formula (1) and formula (2) and :
[0064] , (1)
[0065] , (2)
[0066] said Represents the old policy model, which is used to generate the interaction data between the agent and the environment, and is updated with the latest policy model at regular intervals during the training process; Indicates the strategy model being trained, and continuously updates the parameters of the strategy neural network by using the interaction data obtained during the training process; Indicates the action calculated by the strategy model; s indicates the state of the AUV; N represents a Gaussian distribution, is the covariance matrix of the Gaussian distribution, which is represented by an identity matrix here, is the me...
PUM
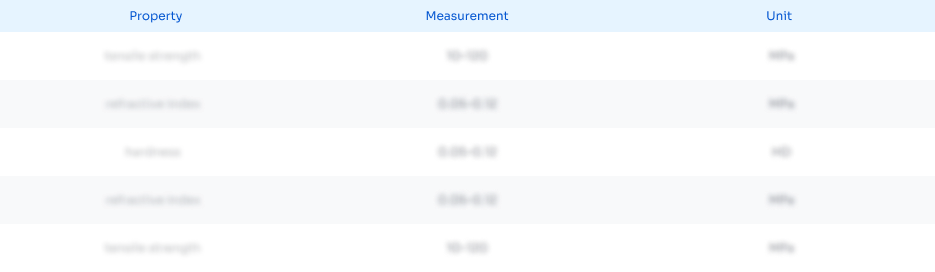
Abstract
Description
Claims
Application Information
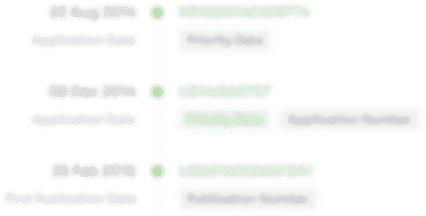
- R&D Engineer
- R&D Manager
- IP Professional
- Industry Leading Data Capabilities
- Powerful AI technology
- Patent DNA Extraction
Browse by: Latest US Patents, China's latest patents, Technical Efficacy Thesaurus, Application Domain, Technology Topic, Popular Technical Reports.
© 2024 PatSnap. All rights reserved.Legal|Privacy policy|Modern Slavery Act Transparency Statement|Sitemap|About US| Contact US: help@patsnap.com