Brain network clustering method based on local attributes and topological structure
A technology of local attributes and topological structures, which is applied to computer components, character and pattern recognition, instruments, etc., and can solve problems such as inaccurate labeling
- Summary
- Abstract
- Description
- Claims
- Application Information
AI Technical Summary
Problems solved by technology
Method used
Image
Examples
Embodiment 1
[0051] ⑴Experimental data
[0052] Taking Alzheimer's disease and normal elderly people as examples, cluster analysis was performed on the brain networks of the two types of subjects; the research data came from the Alzheimer's Disease Neuroimaging Initiative (ADNI) database, including 28 Alzheimer's patients (Age: 72.2±7.5, female: 17, Mini-Mental State Examination (MMSE): 22.8±2.5, Clinical Dementia Rating Scale (CRD): 0.84±0.23), 28 normal elderly people (age: 74.3±6.3 , female: 17, MMSE: 28.9±1.3, CRD: 0±0);
[0053] ⑵Experimental process
[0054] according to figure 1 In the process shown, the following steps are used to cluster the brain networks of 56 subjects:
[0055] Step S1: Preprocessing the fMRI images of the brain, then segmenting the brain regions, and extracting the average time series of each brain region;
[0056] Dparsf software was used to preprocess the functional magnetic resonance images of the brain. The preprocessing steps specifically included: re...
Embodiment 2
[0093] The weight value of step 3 in embodiment 1 is taken as 0.7, and each similarity obtained for step 3: S Atr (G,H),S Str (G,H) and S(G,H) are clustered using a clustering algorithm, and the precision rate, recall rate and F1 value of the clustering results are calculated and tested;
[0094] The precision rate, recall rate and F1 value of each clustering result are as follows: figure 2 shown by figure 2 It can be seen that the weighted combination of local attribute similarity and topology similarity has the best clustering effect of brain network similarity, with a precision rate of 0.64, a recall rate of 0.62, and an F1 of 0.63.
PUM
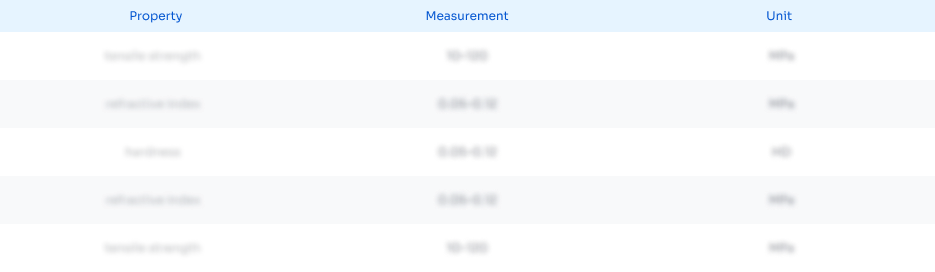
Abstract
Description
Claims
Application Information
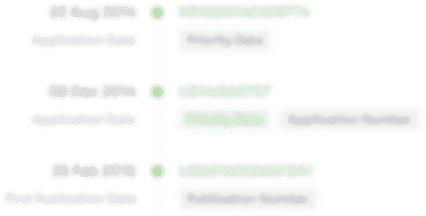
- Generate Ideas
- Intellectual Property
- Life Sciences
- Materials
- Tech Scout
- Unparalleled Data Quality
- Higher Quality Content
- 60% Fewer Hallucinations
Browse by: Latest US Patents, China's latest patents, Technical Efficacy Thesaurus, Application Domain, Technology Topic, Popular Technical Reports.
© 2025 PatSnap. All rights reserved.Legal|Privacy policy|Modern Slavery Act Transparency Statement|Sitemap|About US| Contact US: help@patsnap.com