A phase recovery detection system for intermediate frequency errors of optical components enhanced by convolutional neural network prior
A convolutional neural network and optical element technology, applied in the field of priori enhanced intermediate frequency error phase recovery detection system and optical element intermediate frequency error phase recovery detection system, can solve the problem of low accuracy of surface shape results, failure to converge, and phase recovery detection Problems such as long running time of the system to achieve the effect of improving the convergence performance and speed of convergence
- Summary
- Abstract
- Description
- Claims
- Application Information
AI Technical Summary
Problems solved by technology
Method used
Image
Examples
Embodiment Construction
[0024] The present invention will be described in further detail below in conjunction with the accompanying drawings and specific embodiments.
[0025] The present invention provides a phase recovery detection system for intermediate frequency errors of optical elements enhanced by convolutional neural networks, including a laser 1, a spatial filter 2, a beam expander 3, a linear polarizer 4, an analyzer 5, and a beam splitter mirror 6, total reflection mirror 7, spatial light modulator 8, telescope imaging system 9 and imaging camera 10; the realization process of this system includes three parts: spatial light modulator calibration, training data collection and intermediate frequency error detection.
[0026] Such as figure 1Shown is a schematic diagram of the calibration of the spatial light modulator of the present invention. The laser light emitted by the laser 1 passes through the spatial filter 2 and the beam expander 3 in turn, becomes parallel light, projects on the ...
PUM
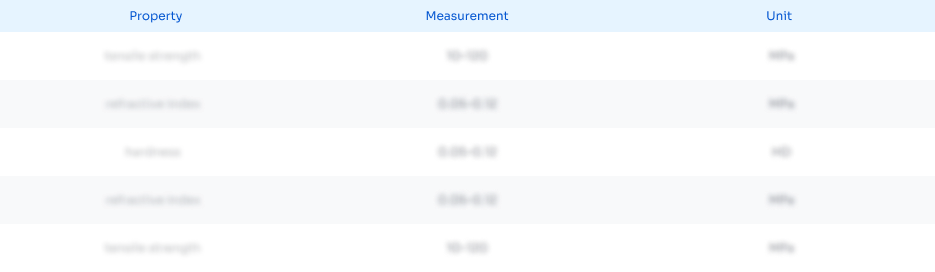
Abstract
Description
Claims
Application Information
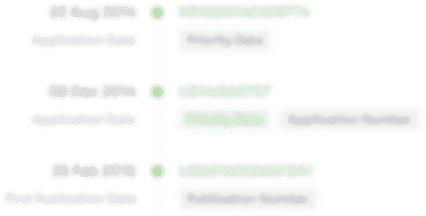
- R&D
- Intellectual Property
- Life Sciences
- Materials
- Tech Scout
- Unparalleled Data Quality
- Higher Quality Content
- 60% Fewer Hallucinations
Browse by: Latest US Patents, China's latest patents, Technical Efficacy Thesaurus, Application Domain, Technology Topic, Popular Technical Reports.
© 2025 PatSnap. All rights reserved.Legal|Privacy policy|Modern Slavery Act Transparency Statement|Sitemap|About US| Contact US: help@patsnap.com