A short-term power load forecasting method based on emd-gru based on feature selection
A short-term power load and feature selection technology, applied in forecasting, electrical components, circuit devices, etc., can solve problems such as large forecasting workload and random errors
- Summary
- Abstract
- Description
- Claims
- Application Information
AI Technical Summary
Problems solved by technology
Method used
Image
Examples
Embodiment Construction
[0037] In order to better understand the technical solutions of the present invention, the embodiments of the present invention will be described in detail below in conjunction with the accompanying drawings.
[0038] It should be clear that the described embodiments of the invention are only some of the embodiments of the present invention, not all of them. Based on the embodiments of the present invention, all other embodiments obtained by persons of ordinary skill in the art without making creative efforts belong to the protection scope of the present invention.
[0039] The embodiment of the present invention provides a kind of EMD-GRU prediction method based on feature selection, refer to figure 1 , which is a schematic flow chart of the EMD-GRU prediction method based on feature selection proposed in the embodiment of the present invention, such as figure 1 As shown, the method includes the following steps:
[0040] Step 101, use the empirical mode decomposition method t...
PUM
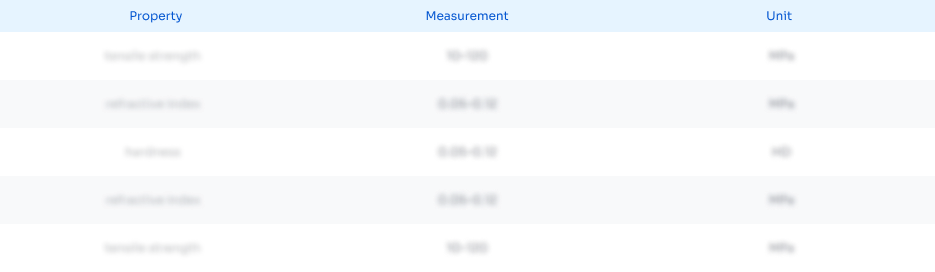
Abstract
Description
Claims
Application Information
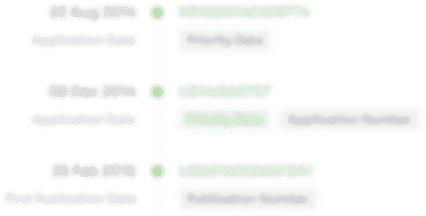
- R&D
- Intellectual Property
- Life Sciences
- Materials
- Tech Scout
- Unparalleled Data Quality
- Higher Quality Content
- 60% Fewer Hallucinations
Browse by: Latest US Patents, China's latest patents, Technical Efficacy Thesaurus, Application Domain, Technology Topic, Popular Technical Reports.
© 2025 PatSnap. All rights reserved.Legal|Privacy policy|Modern Slavery Act Transparency Statement|Sitemap|About US| Contact US: help@patsnap.com