Tongue image texture quantitative analysis method based on multi-scale convolutional neural network
A convolutional neural network and quantitative analysis technology, applied in the field of quantitative analysis of tongue image texture based on multi-scale convolutional neural network, can solve the problems of difficult judgment and low judgment accuracy for doctors, and achieve enhanced pixel recognition ability and exclusive Strong performance and improved detection ability
- Summary
- Abstract
- Description
- Claims
- Application Information
AI Technical Summary
Benefits of technology
Problems solved by technology
Method used
Image
Examples
Embodiment 1
[0084] Example 1: Consistency verification of detection results for small-scale prick targets
[0085] Experimental results such as Figure 4Shown: the present invention extracts 5,000 prick-like tongue images from the tongue image library through random collection of tongue images and expert review, and establishes a test data set according to four categories: point, prick, petechiae and ecchymosis, and no prick. Three methods of image algorithm, MaskRCNN instance segmentation network and multi-scale convolutional neural network are used to detect tongue-like feature items. The detection rate of the multi-scale convolutional neural network algorithm is higher than 80%, and the contour of the detected point and the data marked point overlap by more than 80%, and the situation of missed detection and false detection is significantly lower than other algorithms; tongue surface The analysis of the experimental results of the small-scale feature detection results of pricks is sho...
Embodiment 2
[0090] Example 2: Consistency verification of detection results for medium-scale targets such as tooth marks
[0091] Experimental results such as Figure 5 As shown, the item of the present invention extracts 5000 cases of tooth marks and no tooth marks from the tongue image database through random collection of tongue images and expert review, according to mild tooth marks, moderate tooth marks, severe tooth marks, and no tooth marks Four classes build test datasets. Using three methods of image algorithm, MaskRCNN instance segmentation network and multi-scale convolutional neural network, the tongue and face tooth mark feature item detection experiment is carried out. The detection rate of the multi-scale convolutional neural network algorithm is higher than 85%, and the detected tooth marks and the contours of the tooth marks marked in the data overlap by more than 80%, and the cases of missed detection and false detection are significantly lower than other algorithms; T...
Embodiment 3
[0096] Example 3: Verification of the consistency of detection results for cracked multi-scale targets
[0097] Experimental results such as Figure 6 As shown, the present invention extracts 5,000 cases of cracked and non-cracked tongue images from the tongue image library through randomly collected tongue images and expert reviews, and establishes test data sets according to four categories: mild cracks, moderate cracks, severe cracks, and no cracks. . Three methods of image algorithm, MaskRCNN instance segmentation network and multi-scale convolutional neural network are used to detect the feature items of tongue surface cracks. The detection rate of the multi-scale convolutional neural network algorithm is higher than 85%, and the detected cracks coincide with the contours of the cracks marked in the data by more than 80%, and the cases of missed detection and false detection are significantly lower than other algorithms; The analysis of the experimental results of the m...
PUM
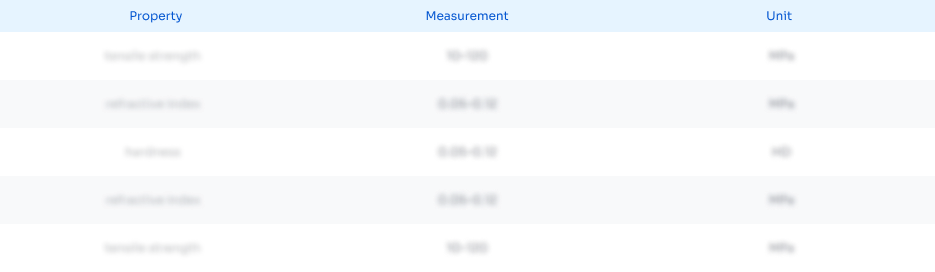
Abstract
Description
Claims
Application Information
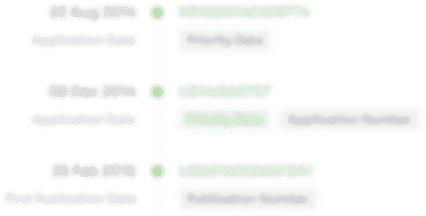
- R&D
- Intellectual Property
- Life Sciences
- Materials
- Tech Scout
- Unparalleled Data Quality
- Higher Quality Content
- 60% Fewer Hallucinations
Browse by: Latest US Patents, China's latest patents, Technical Efficacy Thesaurus, Application Domain, Technology Topic, Popular Technical Reports.
© 2025 PatSnap. All rights reserved.Legal|Privacy policy|Modern Slavery Act Transparency Statement|Sitemap|About US| Contact US: help@patsnap.com