A Motion Recognition Method Based on Feature Selection
A motion recognition and feature selection technology, applied in the field of motion recognition, can solve the problems of low motion recognition efficiency and low motion recognition accuracy, and achieve the effect of reducing feature extraction time, reducing feature dimensions, and improving efficiency
- Summary
- Abstract
- Description
- Claims
- Application Information
AI Technical Summary
Problems solved by technology
Method used
Image
Examples
specific Embodiment approach 1
[0018] Specific implementation mode one: as figure 1 As shown, a kind of motion recognition method based on feature selection described in this embodiment, the method comprises the following steps:
[0019] Step 1, separately for the M of the human body 0 Each action is used for data collection, and when collecting the data of each action, a three-axis accelerometer, a three-axis gyroscope and a three-axis magnetometer are used simultaneously. M 0 The number of action types contained in an action is N 0 ;
[0020] The collected three-axis accelerometer data, three-axis gyroscope data, three-axis magnetometer data and three-axis attitude angle data (the three-axis attitude angle data is collected by the three-axis gyroscope) as raw data;
[0021] Preprocess the original data to obtain the preprocessed data, and perform action interception on the preprocessed data to obtain action segment data, wherein: each action corresponds to an action segment data;
[0022] Action segme...
specific Embodiment approach 2
[0031] Specific embodiment 2: The difference between this embodiment and specific embodiment 1 is that the statistical features include mean value feature, standard deviation feature, maximum value feature, minimum value feature, median absolute deviation feature, and interquartile range feature and related features;
[0032] The signal time-frequency features include energy average features, signal amplitude area features, signal entropy features, maximum magnitude amplitude features, maximum magnitude frequency features, secondary maximum magnitude amplitude features, secondary maximum magnitude frequency features, average normalization Frequency characteristics, amplitude distribution kurtosis characteristics and amplitude distribution skewness characteristics;
[0033] The complex modeling features include autoregressive coefficient features and Mel frequency cepstrum features.
specific Embodiment approach 3
[0034] Specific implementation mode three: the difference between this implementation mode and specific implementation mode two is: the feature in step two is screened by using the number of division index and the information gain index, and the specific process is as follows:
[0035] Divide times index
[0036] The basic model of the extreme gradient boosting tree model is a classification regression tree. The direction of the data at the branch node is determined by the relationship between its feature value and the threshold value. The number of times a feature is selected to achieve splitting directly reflects the feature in the model decision. A way to evaluate the importance of features is to use the sum of the number of times the feature variable is used for division in all decision tree models as the score;
[0037] Note that the extreme gradient boosting tree model integrates a total of Z decision trees, and the feature value of a certain dimension x i In the decisi...
PUM
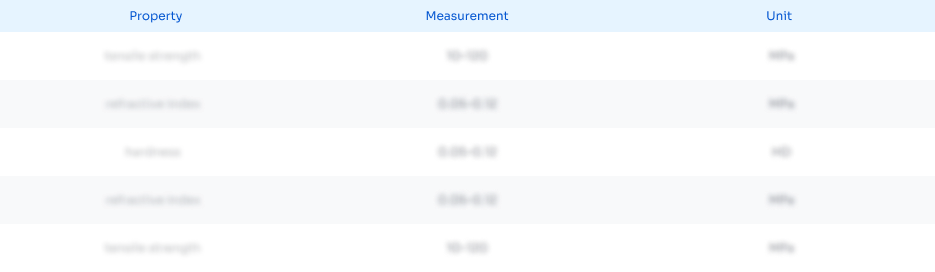
Abstract
Description
Claims
Application Information
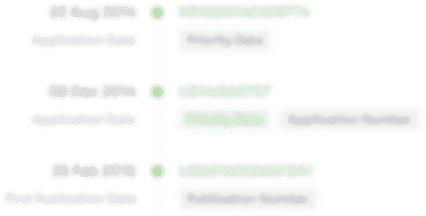
- R&D Engineer
- R&D Manager
- IP Professional
- Industry Leading Data Capabilities
- Powerful AI technology
- Patent DNA Extraction
Browse by: Latest US Patents, China's latest patents, Technical Efficacy Thesaurus, Application Domain, Technology Topic, Popular Technical Reports.
© 2024 PatSnap. All rights reserved.Legal|Privacy policy|Modern Slavery Act Transparency Statement|Sitemap|About US| Contact US: help@patsnap.com