Motion recognition method based on feature selection
A motion recognition and feature selection technology, applied in the field of motion recognition, can solve the problems of low motion recognition accuracy and low motion recognition efficiency, and achieve the effect of reducing feature extraction time, reducing feature dimensions, and improving efficiency
- Summary
- Abstract
- Description
- Claims
- Application Information
AI Technical Summary
Problems solved by technology
Method used
Image
Examples
specific Embodiment approach 1
[0018] Specific implementation manner one: such as figure 1 As shown, the motion recognition method based on feature selection described in this embodiment includes the following steps:
[0019] Step one: separate M on the human body 0 Data collection for each action, and when collecting data for each action, a three-axis accelerometer, a three-axis gyroscope, and a three-axis magnetometer are used simultaneously. M 0 The number of action types included in each action is N 0 ;
[0020] Use the collected three-axis accelerometer data, three-axis gyroscope data, three-axis magnetometer data, and three-axis attitude angle data (three-axis attitude angle data is collected by a three-axis gyroscope) as raw data;
[0021] Preprocess the original data to obtain the preprocessed data, and perform action interception on the preprocessed data to obtain the action segment data, where: each action corresponds to one action segment data;
[0022] Action segment data X for an action N×M , X N×M ...
specific Embodiment approach 2
[0031] Specific embodiment two: this embodiment is different from specific embodiment one in that the statistical features include mean feature, standard deviation feature, maximum feature, minimum feature, median absolute deviation feature, interquartile feature And related features;
[0032] The signal time-frequency characteristics include energy mean value characteristics, signal amplitude area characteristics, signal entropy characteristics, maximum magnitude amplitude characteristics, maximum magnitude frequency characteristics, secondary maximum magnitude amplitude characteristics, secondary maximum magnitude frequency characteristics, and average normalization Frequency characteristics, kurtosis characteristics of amplitude distribution, and skewness characteristics of amplitude distribution;
[0033] The complex modeling features include autoregressive coefficient features and Mel frequency cepstrum features.
specific Embodiment approach 3
[0034] Specific embodiment three: This embodiment is different from the specific embodiment two in that: the division number index and the information gain index are used to screen the features in step two, and the specific process is:
[0035] Division index
[0036] The basic model of the extreme gradient boosting tree model is the classification regression tree. The direction of the data at the branch node is determined by the relationship between its feature value and the threshold. The number of times a feature is selected to achieve split directly reflects the feature in the model decision. The size of the role of, a way of evaluating the importance of features is to score the sum of the number of times that feature variables are used for division in all decision tree models;
[0037] The extreme gradient boosting tree model integrates a total of Z decision trees, a certain dimension eigenvalue x i In decision tree F j Is used for decision making in C j , Where: i=1, 2,...,462,...
PUM
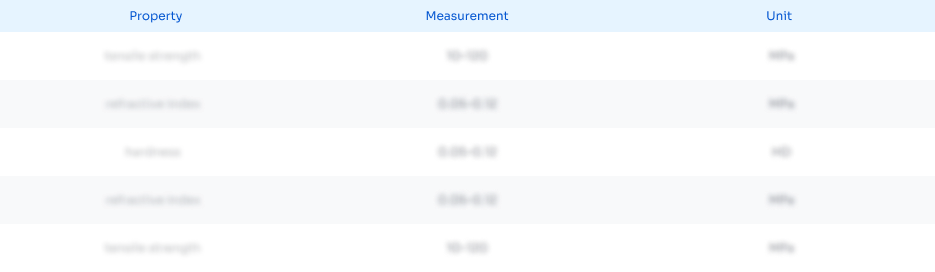
Abstract
Description
Claims
Application Information
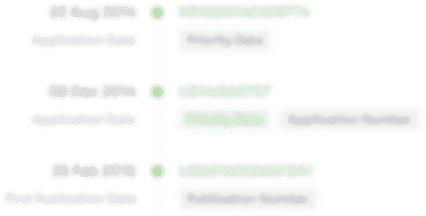
- R&D Engineer
- R&D Manager
- IP Professional
- Industry Leading Data Capabilities
- Powerful AI technology
- Patent DNA Extraction
Browse by: Latest US Patents, China's latest patents, Technical Efficacy Thesaurus, Application Domain, Technology Topic, Popular Technical Reports.
© 2024 PatSnap. All rights reserved.Legal|Privacy policy|Modern Slavery Act Transparency Statement|Sitemap|About US| Contact US: help@patsnap.com