Social relation knowledge graph generation method based on artificial intelligence and robot system
A technology of knowledge graph and relational knowledge, applied in relational databases, instruments, semantic tool creation, etc., can solve problems such as lack of comprehensive consideration of time and space
- Summary
- Abstract
- Description
- Claims
- Application Information
AI Technical Summary
Problems solved by technology
Method used
Image
Examples
Embodiment 1
[0072] Such as figure 1 As shown, a method for generating a social relationship knowledge graph is provided, including the step S100 of acquiring experience, the step S200 of extracting experience, the step S300 of intersecting experience, the step S400 of acquiring intersection information, and the step S500 of generating relationship.
[0073] The experience obtaining step S100 is used to obtain the experience in each user's resume. The resume includes the user's personal resume, also includes resumes obtained from network information such as social networking sites, and also includes all information including the user's experience.
[0074] The experience extraction step S200 is used to extract the time period of each experience and the unit where the user is located in the time period from the experience of each user's resume.
[0075] Experience intersection step S300, for seeking the intersection of every two experiences belonging to different users
[0076] E.g:
[0...
Embodiment 2
[0094] Such as figure 2 As shown, according to the social relationship knowledge map generation method provided in Example 1,
[0095] Wherein, the experience acquisition step S100 includes the education and work experience acquisition step S110.
[0096] Education and work experience acquisition step S110 is used to acquire the education experience and work experience in each user's resume.
[0097] The resume can be input by the user, or obtained from Baidu Encyclopedia or other websites, and the education experience and work experience can be extracted from it.
[0098] E.g
[0099] Zhang San
[0100] Educational experience
[0101] 2010.9-2014.7 A1 University B11 College
[0102] 2014.9-2017.7 A2 University B21 College
[0103] work experience
[0104] 2017.9-2018.7 A3 company B31 department
[0105] 2018.9-2019.7 A4 company B41 department
[0106] Li Si
[0107] Educational experience
[0108] 2011.9-2015.7 A5 University B51 College
[0109] 2015.9-2018.7 A2 ...
Embodiment 3
[0131] Such as image 3 As shown, according to the social relationship knowledge map generation method provided in Example 1,
[0132] Wherein, the intersection information obtaining step S400 includes a first intersection information obtaining step S410.
[0133] The first intersection information acquisition step S410 is used to acquire the time period and unit information of the intersection part for every two experiences belonging to different users whose time period intersection is not empty and unit intersection is not empty by matching the experiences.
[0134] The specific steps for finding the unit intersection of two user experiences (one experience of user A and one experience of user B)
[0135] Extract first-level unit names (such as school names, company names, and research institute names, identified and extracted based on keywords such as "university, company, research institute"), and second-level unit names (such as college names) from the unit information e...
PUM
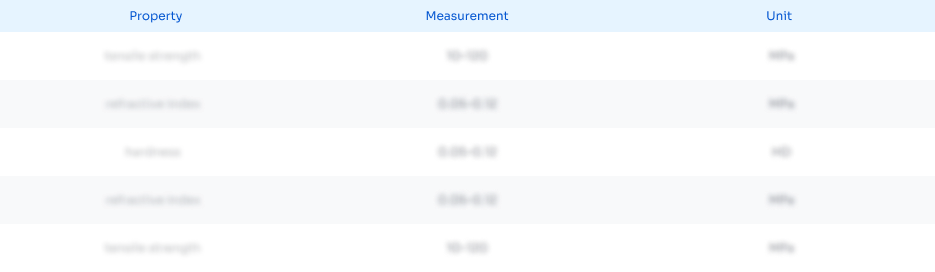
Abstract
Description
Claims
Application Information
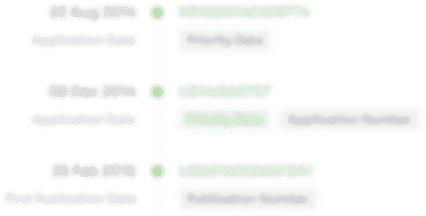
- R&D Engineer
- R&D Manager
- IP Professional
- Industry Leading Data Capabilities
- Powerful AI technology
- Patent DNA Extraction
Browse by: Latest US Patents, China's latest patents, Technical Efficacy Thesaurus, Application Domain, Technology Topic, Popular Technical Reports.
© 2024 PatSnap. All rights reserved.Legal|Privacy policy|Modern Slavery Act Transparency Statement|Sitemap|About US| Contact US: help@patsnap.com