Object detection device, object detection method, and program
A technology of object detection and detection algorithm, which is applied in biometrics recognition, image data processing, instruments, etc., can solve the problem that the deep learning algorithm is not easy, and achieve the effect of high-speed and high-precision object detection
- Summary
- Abstract
- Description
- Claims
- Application Information
AI Technical Summary
Problems solved by technology
Method used
Image
Examples
no. 1 approach
[0045] Embodiments described below relate to a face detection device (object detection device) that is mounted on a portable information terminal such as a smartphone and detects a face from an image. However, this is just an example, and the object detected from the image does not necessarily have to be a face, and may be any object. In addition, the face detection device (object detection device) may be mounted on any information processing device (computer) other than the portable information terminal.
[0046] The face detection device of the present embodiment includes a first face detection unit using Haar-like feature values and adaboost, and a second face detection unit using deep learning. However, if deep learning-type face detection is performed on the entire input image, the processing time becomes very long. Therefore, the face detection device of this embodiment performs detection based on the first face detection unit on the entire input image to determine ar...
no. 2 approach
[0095] In the first embodiment, a case where face detection is performed on one image has been described. In this embodiment, face detection is continuously performed on a plurality of frame images constituting a moving image. The face detection processing for each frame image is basically the same as that of the first embodiment, but the integration region creation processing ( figure 2 Step S3) of different. Since the same object exists at the same position in consecutive frame images, in this embodiment, when determining the integration area, information about the current frame and information about past frames are taken into consideration.
[0096] Several methods are conceivable as a method of determining a specific integration area. For example, a method is assumed in which an integration area (hereinafter referred to as a tentative integration area) is determined by the same method as in the first embodiment for the current frame and the past frame (the latest frame)...
no. 3 approach
[0101] In the first embodiment, the second detection unit 114 performs face detection in consideration of only the image information (pixel values) of the integration area. The second detection unit 114 of the present embodiment also receives the detection result of the first detection unit 112 related to the integration area as an input, and performs face detection processing. The “detection result of the first detection unit 112 related to the integrated area” includes face orientation, age, gender, race, expression, and the like.
[0102] In order to enable the second detection unit 114 to perform such recognition, in the learning process (generation process) of the second detection unit 114, the image data including the image data and the detection results (face orientation, age, etc.) of the image data obtained by the first detection unit 112 are used. , gender, race, expression, etc.) learning data.
[0103] In this way, by performing detection processing using the dete...
PUM
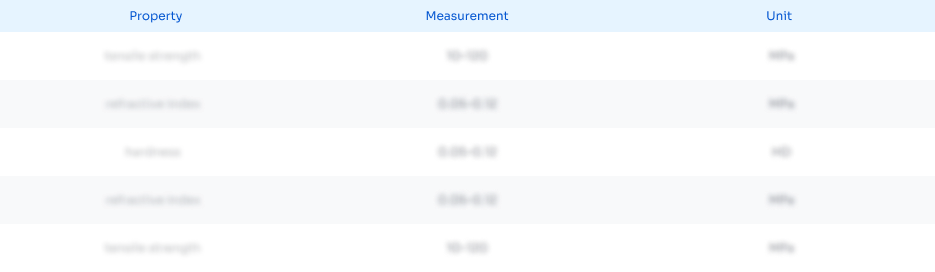
Abstract
Description
Claims
Application Information
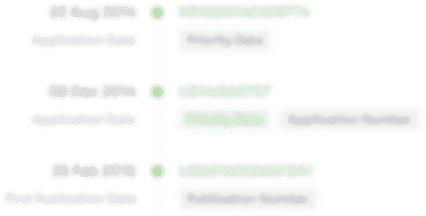
- R&D Engineer
- R&D Manager
- IP Professional
- Industry Leading Data Capabilities
- Powerful AI technology
- Patent DNA Extraction
Browse by: Latest US Patents, China's latest patents, Technical Efficacy Thesaurus, Application Domain, Technology Topic, Popular Technical Reports.
© 2024 PatSnap. All rights reserved.Legal|Privacy policy|Modern Slavery Act Transparency Statement|Sitemap|About US| Contact US: help@patsnap.com