Sample imbalance-oriented multi-disease classifier design method
A disease classification and design method technology, applied in the direction of instruments, calculations, computer components, etc., can solve problems such as unbalanced data sets, and achieve the effect of small feature subsets and good effects
- Summary
- Abstract
- Description
- Claims
- Application Information
AI Technical Summary
Problems solved by technology
Method used
Image
Examples
Embodiment Construction
[0034] In order to deepen understanding of the present invention, below will combine existing method and attached figure 1 The present invention is described in further detail. Existing methods are only used to explain the present invention, and do not constitute a limitation to the protection scope of the present invention.
[0035] This application is a multi-disease classification method for sample imbalance, the specific process is as follows figure 1 As shown, including the following five aspects:
[0036] Step 1, divide the unbalanced samples into sample subsets according to their disease categories;
[0037] Step 2, feature selection based on disease association rules;
[0038] Step 3, random iterative equalization sampling based on the unbalance degree as the upper limit;
[0039] Step 4, train the weak classifier and calculate the classification effect;
[0040] Step 5, complete the disease classification prediction by judging whether the difference of macro-F1 me...
PUM
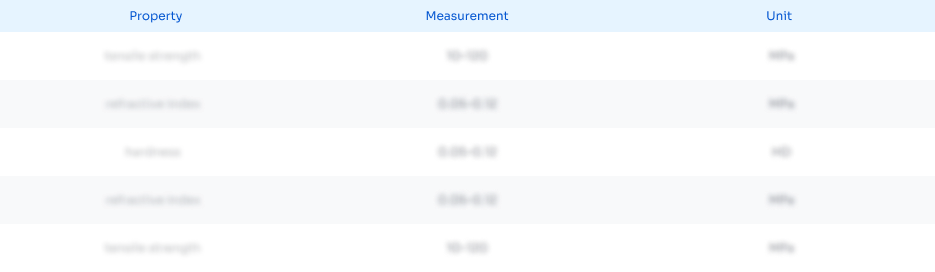
Abstract
Description
Claims
Application Information
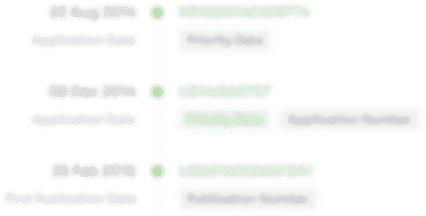
- R&D
- Intellectual Property
- Life Sciences
- Materials
- Tech Scout
- Unparalleled Data Quality
- Higher Quality Content
- 60% Fewer Hallucinations
Browse by: Latest US Patents, China's latest patents, Technical Efficacy Thesaurus, Application Domain, Technology Topic, Popular Technical Reports.
© 2025 PatSnap. All rights reserved.Legal|Privacy policy|Modern Slavery Act Transparency Statement|Sitemap|About US| Contact US: help@patsnap.com