A multi-task-assisted extreme multi-label short text classification method using co-occurrence information
A text classification and classification method technology, applied in the information field, can solve the problems of effect dependence, difficult to apply effectively, increase the number of neurons in the output layer, etc., achieve the effect of reducing maintenance costs, solving high maintenance costs, and enhancing robustness
- Summary
- Abstract
- Description
- Claims
- Application Information
AI Technical Summary
Problems solved by technology
Method used
Image
Examples
Embodiment Construction
[0034] The present invention will be described in further detail below in conjunction with the accompanying drawings.
[0035] The design idea of the present invention is to use co-occurrence information and multi-task learning technology to assist in improving the prediction effect of extreme multi-label text classification tasks. Inspired by the explicit association relationship between co-occurrence information and tags in the tag set, the explicit association relationship inspires the present invention to construct co-occurrence information by using the relevant feature information of the account itself, thereby effectively improving the prediction effect of the method on high-frequency tags and low-frequency tags. Further, inspired by the shared parameters in multi-task learning, the present invention uses the information learned from the multi-label text classification task to assist the extreme multi-label text classification task when it is difficult to simply solve p...
PUM
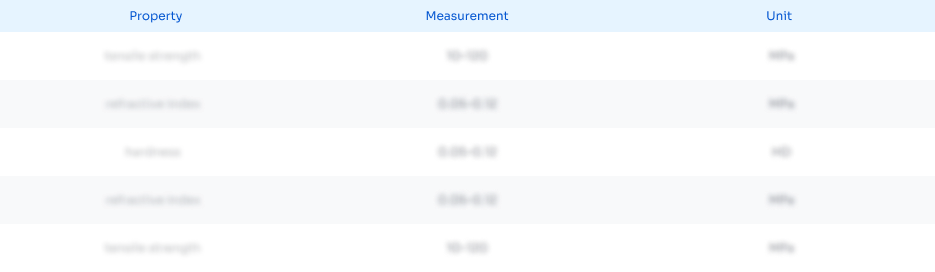
Abstract
Description
Claims
Application Information
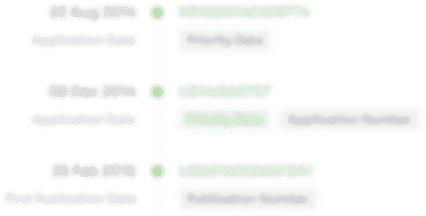
- R&D Engineer
- R&D Manager
- IP Professional
- Industry Leading Data Capabilities
- Powerful AI technology
- Patent DNA Extraction
Browse by: Latest US Patents, China's latest patents, Technical Efficacy Thesaurus, Application Domain, Technology Topic, Popular Technical Reports.
© 2024 PatSnap. All rights reserved.Legal|Privacy policy|Modern Slavery Act Transparency Statement|Sitemap|About US| Contact US: help@patsnap.com