Reservoir evaluation model construction method and reservoir identification method
A technology for evaluating models and construction methods, applied in the fields of artificial intelligence and geophysical exploration, can solve the problems of lack of sample support, lack of reservoir identification sample data, low coincidence rate, etc., to improve utilization efficiency, improve accuracy and efficiency, The effect of improving the matching rate
- Summary
- Abstract
- Description
- Claims
- Application Information
AI Technical Summary
Problems solved by technology
Method used
Image
Examples
Embodiment 1
[0053] Taking the oil test data and logging data of a certain oil well as an example, the present invention is further described,
[0054] 1. Table 1 is the logging data table of the starting and ending depth intervals of the oil testing data collected in a well target formation in a certain research area of Zhongyuan Oilfield. The oil testing results of this depth section are oil layers. It can be seen from the table that the logging data includes Medium-induction RILM, deep-induction RILD, compensated density DEN, compensated neutron CNL and compensated acoustic AC data; the logging data are actually continuous data, and Table 1 only gives some of them.
[0055] Table 1
[0056]
[0057]
[0058] 2. The well logging data (continuous data) determined in Table 1 is divided into the data of a depth point row by depth, forming the logging row data of a single depth point as shown in Table 2;
[0059] Table 2
[0060]
[0061]
[0062] 3. Sort the logging line dat...
Embodiment 2
[0078] In this embodiment, the process of expanding the capacity of a small sample is taken as an example to further explain the solution of this application within the start and end depths of oil testing data in a target formation of a certain well in a certain research area, and when the oil testing result is a water layer.
[0079] 1) Table 7 is the well logging data table after standardization of the starting and ending depth intervals of the oil testing data collected in the target formation of a certain well in a certain research area. The oil testing results of this depth section are water layers. Includes medium induction, deep induction, compensated density, compensated neutron and compensated acoustic data;
[0080] Table 7
[0081]
[0082] 2) The logging data determined in Table 7 is divided into data of a row of depth points according to depth, forming the logging row data of a single depth point as shown in Table 8;
[0083] Table 8
[0084]
[0085]
...
Embodiment 3
[0102] The difference between this embodiment and the above-mentioned specific embodiments only lies in:
[0103] In this embodiment, there is no need to sort the logging row data, and the logging row data of the set data are randomly extracted. Through this random sampling method, the efficiency of sample expansion is effectively improved.
PUM
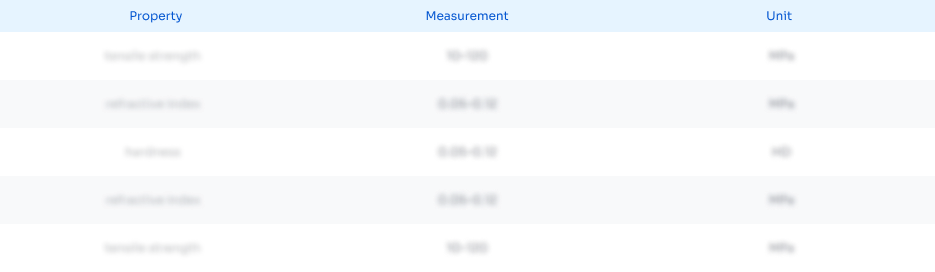
Abstract
Description
Claims
Application Information
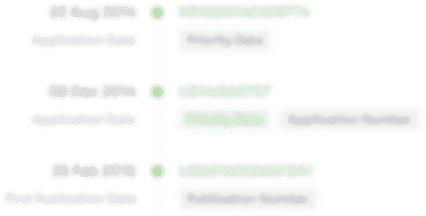
- R&D Engineer
- R&D Manager
- IP Professional
- Industry Leading Data Capabilities
- Powerful AI technology
- Patent DNA Extraction
Browse by: Latest US Patents, China's latest patents, Technical Efficacy Thesaurus, Application Domain, Technology Topic.
© 2024 PatSnap. All rights reserved.Legal|Privacy policy|Modern Slavery Act Transparency Statement|Sitemap