A Method for Bus Travel Time Prediction Based on Sparse GPS Data
A GPS data and travel time technology, applied in forecasting, data processing applications, traffic flow detection, etc., can solve the problem of low reliability of bus travel time prediction, achieve fast travel services, improve error accuracy, and have good application prospects. Effect
- Summary
- Abstract
- Description
- Claims
- Application Information
AI Technical Summary
Problems solved by technology
Method used
Image
Examples
specific Embodiment approach 1
[0020] Specific implementation mode one: combine figure 1 Illustrate this embodiment, the concrete process of a kind of bus travel time prediction method based on sparse GPS data in this embodiment is:
[0021] Step 1. After receiving the original GPS data uploaded by the public transport vehicle, carry out normalized preprocessing to the original GPS data of the public transport, and integrate the data after normalized preprocessing into a two-dimensional space-time velocity matrix (as described in step 2 model input);
[0022] Step 2. Construct a generative confrontation network based on traffic flow prior rules to generate traffic conditions that fit the actual bus line;
[0023] Step 3. Based on the model-free adaptive driving method, the bus travel time is predicted online.
specific Embodiment approach 2
[0024] Specific embodiment two: the difference between this embodiment and the specific embodiment one is that after receiving the original GPS data uploaded by the public transport vehicle in the step one, normalization preprocessing is carried out to the original GPS data of the public transport, and normalized The preprocessed data are integrated into a two-dimensional space-time velocity matrix (as the input of the model described in step 2); the specific process is as follows:
[0025] Step 11, after receiving the original GPS data uploaded by the bus vehicle, normalize the original GPS data of the bus and preprocess it; the process is:
[0026] 1) After receiving the original GPS data uploaded by the bus, the data whose speed exceeds the maximum speed limit of the bus (50km / h) is regarded as an abnormal value and deleted;
[0027] 2) In order to avoid the interference of different magnitudes of original data on network learning, normalize the original GPS data uploaded b...
specific Embodiment approach 3
[0034] Specific embodiment three: the difference between this embodiment and specific embodiment one or two is that in the step two, a generative confrontation network based on traffic flow prior rules is constructed to generate a traffic state of a bus line that fits the reality; the specific process is:
[0035] The present invention uses the bus speed as an index to measure the traffic state of the bus line. After receiving the processed bus GPS data, it is necessary to generate the state of the empty value position in the speed matrix, so as to obtain the complete bus traffic status and provide it to step 3 for bus Travel time forecast. In conventional data generation algorithms, an encoding-decoding structure is often used to extract and restore data features, so as to obtain the generated results. However, the gradual feature restoration process will lead to the continuous accumulation of errors, which will affect the accuracy of the output results. The present inventio...
PUM
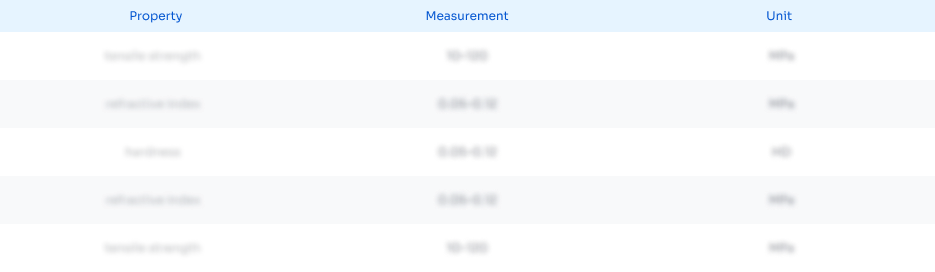
Abstract
Description
Claims
Application Information
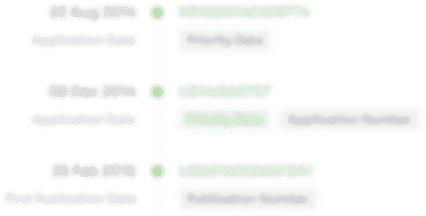
- R&D Engineer
- R&D Manager
- IP Professional
- Industry Leading Data Capabilities
- Powerful AI technology
- Patent DNA Extraction
Browse by: Latest US Patents, China's latest patents, Technical Efficacy Thesaurus, Application Domain, Technology Topic, Popular Technical Reports.
© 2024 PatSnap. All rights reserved.Legal|Privacy policy|Modern Slavery Act Transparency Statement|Sitemap|About US| Contact US: help@patsnap.com