Traffic jam prediction method and system based on convolutional long short-term memory neural network
A long-short-term memory and traffic congestion technology, applied in the field of transportation, can solve the problems of redundant and complex data collection, low accuracy, and insufficient real-time performance, and achieve accurate, efficient, and intuitive prediction results
Active Publication Date: 2021-06-15
SHANGHAI JIAO TONG UNIV
View PDF6 Cites 8 Cited by
- Summary
- Abstract
- Description
- Claims
- Application Information
AI Technical Summary
Problems solved by technology
For the existing short-term traffic congestion prediction methods, the accuracy is not high, the real-time performance is not en
Method used
the structure of the environmentally friendly knitted fabric provided by the present invention; figure 2 Flow chart of the yarn wrapping machine for environmentally friendly knitted fabrics and storage devices; image 3 Is the parameter map of the yarn covering machine
View moreImage
Smart Image Click on the blue labels to locate them in the text.
Smart ImageViewing Examples
Examples
Experimental program
Comparison scheme
Effect test
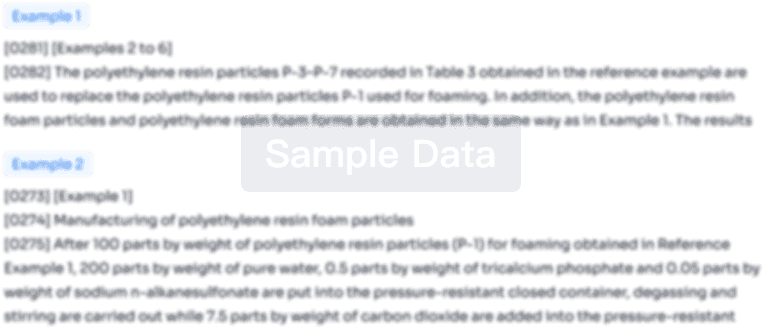
PUM
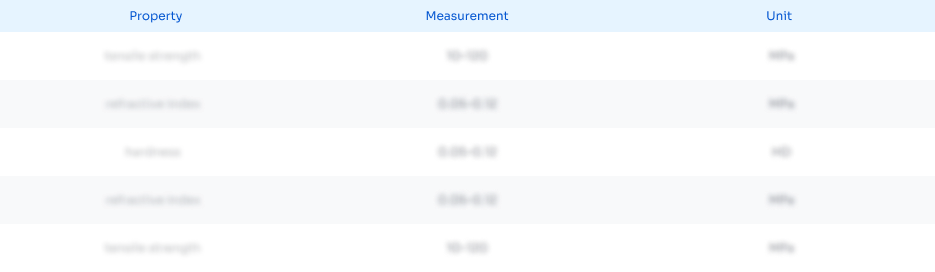
Abstract
The invention relates to a traffic jam prediction method based on a convolutional long short-term memory neural network. The method comprises the following steps: S1, acquiring historical information related to traffic jam; S2, constructing a traffic jam index based on historical information; S3, constructing a prediction model based on a convolutional neural network and a bidirectional long-short time memory neural network; S4, training a prediction model based on the traffic jam indexes; S5, predicting the traffic jam condition in the future time period through the prediction model based on the historical information. In addition, the invention also discloses a traffic jam prediction system based on the convolutional long short-term memory neural network. According to the method, a reasonable and visual traffic jam index is constructed based on the traffic speed, and the time, space and periodic characteristics of the traffic data are fully extracted by using the convolutional neural network and the bidirectional long-short-term memory network, so that the fitting degree of the output prediction result and the actual traffic jam condition is greatly improved.
Description
technical field [0001] The present invention relates to the technical field of transportation, in particular to a prediction method for predicting traffic conditions, in particular to a traffic congestion prediction method based on convolutional bidirectional long-short-term memory neural network. Background technique [0002] Traffic congestion has seriously affected people's quality of life. Even though the traffic management department has introduced various mitigation policies, with the explosive growth of the number of motor vehicles, traffic congestion is still one of the most important traffic problems we should pay attention to at present. More accurate short-term traffic congestion prediction can not only provide reliable management basis for traffic managers, but also show the actual road status to road users, which is convenient for them to make judgments, and plays a huge role in alleviating traffic congestion and reducing traffic accidents. effect. [0003] Sho...
Claims
the structure of the environmentally friendly knitted fabric provided by the present invention; figure 2 Flow chart of the yarn wrapping machine for environmentally friendly knitted fabrics and storage devices; image 3 Is the parameter map of the yarn covering machine
Login to View More Application Information
Patent Timeline
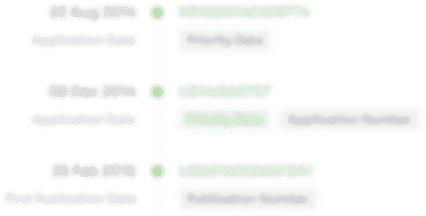
IPC IPC(8): G06Q10/04G06Q10/06G06Q50/30G06F30/27G06N3/04G06N3/08
CPCG06Q10/04G06Q10/06393G06Q50/30G06F30/27G06N3/08G06N3/044G06N3/045Y02T10/40
Inventor 倪安宁李桃俞岑歆陈钦钦
Owner SHANGHAI JIAO TONG UNIV
Features
- R&D
- Intellectual Property
- Life Sciences
- Materials
- Tech Scout
Why Patsnap Eureka
- Unparalleled Data Quality
- Higher Quality Content
- 60% Fewer Hallucinations
Social media
Patsnap Eureka Blog
Learn More Browse by: Latest US Patents, China's latest patents, Technical Efficacy Thesaurus, Application Domain, Technology Topic, Popular Technical Reports.
© 2025 PatSnap. All rights reserved.Legal|Privacy policy|Modern Slavery Act Transparency Statement|Sitemap|About US| Contact US: help@patsnap.com