A method for generating high-quality simulated experiences for dialogue policy learning
A policy learning and high-quality technology, applied in the field of machine learning, can solve the problems of weakening the advantages of Dyna-Q framework and low efficiency of DDQ, so as to avoid poor learning effect
- Summary
- Abstract
- Description
- Claims
- Application Information
AI Technical Summary
Problems solved by technology
Method used
Image
Examples
Embodiment 1
[0054] Such as figure 1 As shown, this scheme proposes a GP-based deep Dyna-Q method for dialogue policy learning. The basic method is consistent with the existing technology, such as using human conversation data to initialize the dialogue policy model and world model, and then Start dialogue policy learning. The dialogue policy learning of the dialogue policy model mainly includes two parts: direct reinforcement learning and indirect reinforcement learning (also called planning). Direct reinforcement learning, using Deep Q-Network (DQN) to improve the dialogue policy based on real experience, the dialogue policy model interacts with the user User, in each step, the dialogue policy model maximizes the value function Q according to the observed dialogue state s, Select the action a to perform. Then, the dialog policy model receives the reward r, the real user's action a r u , and update the current state to s’, and then the real experience (s, a, r, a r u , t) is stored...
Embodiment 2
[0086] Such as Figure 9 As shown, this embodiment is similar to Embodiment 1, and the difference is that in this embodiment, before storing the simulation experience in the buffer, the quality detector performs quality inspection on the simulation experience, and passes the quality inspection. The experience is stored in the buffer.
[0087] Specifically, the upper bound simulation experience e is detected by the quality detector respectively l , lower limit simulation experience e b and meta-simulation experience e i the quality of. The quality detector here can use the traditional GAN (generative confrontation network) quality detector, or the KL divergence (Kullback-Leibler divergence) quality detector independently developed by the applicant.
[0088] The following is a brief introduction to the KL divergence quality detector, such as Figure 4 As shown, the quality inspection of the simulated experience is mainly carried out by comparing the simulated experience w...
PUM
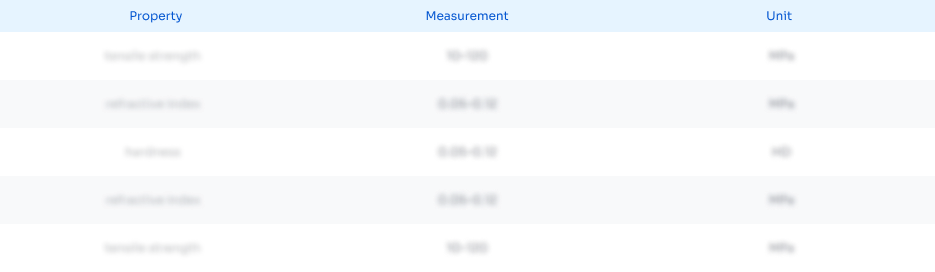
Abstract
Description
Claims
Application Information
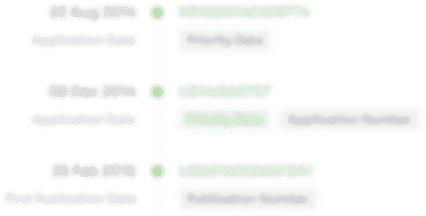
- R&D
- Intellectual Property
- Life Sciences
- Materials
- Tech Scout
- Unparalleled Data Quality
- Higher Quality Content
- 60% Fewer Hallucinations
Browse by: Latest US Patents, China's latest patents, Technical Efficacy Thesaurus, Application Domain, Technology Topic, Popular Technical Reports.
© 2025 PatSnap. All rights reserved.Legal|Privacy policy|Modern Slavery Act Transparency Statement|Sitemap|About US| Contact US: help@patsnap.com