Vehicle real-time overload detection method based on convolutional neural network
A technology of convolutional neural network and detection method, which is applied in the direction of neural learning method, biological neural network model, neural architecture, etc., can solve the problems of artificial inability to work 24 hours, inability to guarantee accuracy, and long time-consuming parking detection. Road non-stop overload detection, avoiding traffic jams and road traffic accidents, good real-time detection effect
- Summary
- Abstract
- Description
- Claims
- Application Information
AI Technical Summary
Problems solved by technology
Method used
Image
Examples
Embodiment Construction
[0041] According to the above description, the following describes the specific implementation process of the present invention.
[0042] The offline part is divided into 2 steps:
[0043] Step 1: Data Acquisition
[0044] Use the camera to collect on-the-spot and shoot from multiple angles and scenes to ensure that the photos of vehicles with each number of axles and wheelbases are covered, and the number is about 5,000.
[0045] Step 1.1: Dataset production
[0046] Label the wheels and body on the photos taken, and make them into a data set in VOC format.
[0047] Step 2: YOLO-V3 network framework construction and model training
[0048] The idea of the YOLO series of algorithms is to use a picture to be detected as input, through a convolutional network, to directly classify and return the bounding box. The network structure of YOLO-V3 (as attached figure 2 Shown) consists of two parts, one is the backbone network Darknet-53 responsible for feature extraction, and ...
PUM
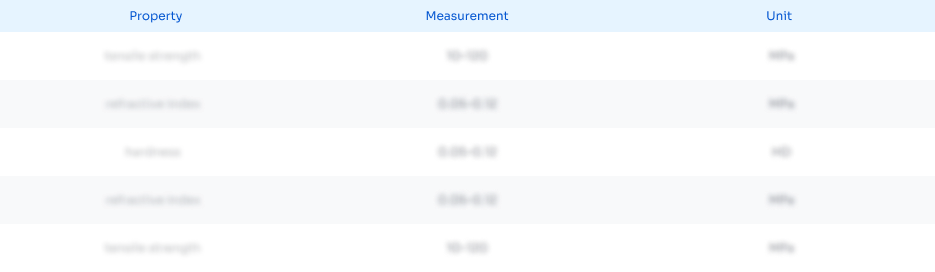
Abstract
Description
Claims
Application Information
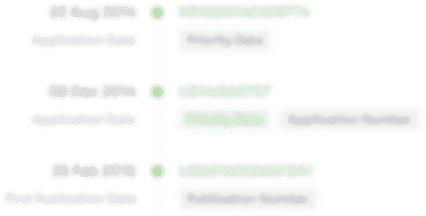
- R&D Engineer
- R&D Manager
- IP Professional
- Industry Leading Data Capabilities
- Powerful AI technology
- Patent DNA Extraction
Browse by: Latest US Patents, China's latest patents, Technical Efficacy Thesaurus, Application Domain, Technology Topic, Popular Technical Reports.
© 2024 PatSnap. All rights reserved.Legal|Privacy policy|Modern Slavery Act Transparency Statement|Sitemap|About US| Contact US: help@patsnap.com