Automatic model evaluation method and device based on batch normalization layer
An automatic evaluation and normalization technology, applied in the field of computer vision, can solve the problems of consuming a lot of manpower and material resources, the model evaluation method is not feasible, and the test data sample size is large, so as to save manpower, material resources and time
- Summary
- Abstract
- Description
- Claims
- Application Information
AI Technical Summary
Problems solved by technology
Method used
Image
Examples
Embodiment Construction
[0033] A batch normalization layer-based model automatic evaluation method and device of the present invention first extracts the batch normalization layer parameters of the fourth module of the model as a benchmark after the first training is completed; then evaluates the model when needed The second training is performed on the unlabeled test data set. During the second training process, the parameters of the non-batch normalization layer do not need to be updated, and the gradient backpropagation is not required. Only the mean and variance of the batch normalization layer need to be updated. Two parameters; after the second training is completed, the batch normalization layer parameters of the fourth module of the model after the second training are also extracted; After the second training, the parameters extracted were normalized by Gaussian respectively, and then the Euclidean distance of the two groups of parameters was calculated, and the distance was used to evaluate t...
PUM
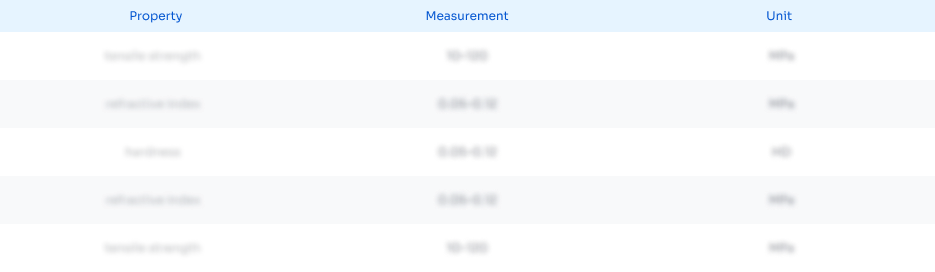
Abstract
Description
Claims
Application Information
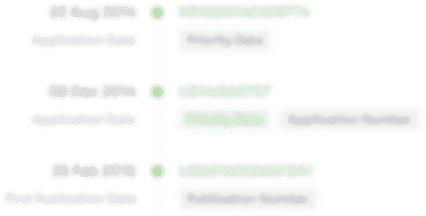
- R&D Engineer
- R&D Manager
- IP Professional
- Industry Leading Data Capabilities
- Powerful AI technology
- Patent DNA Extraction
Browse by: Latest US Patents, China's latest patents, Technical Efficacy Thesaurus, Application Domain, Technology Topic, Popular Technical Reports.
© 2024 PatSnap. All rights reserved.Legal|Privacy policy|Modern Slavery Act Transparency Statement|Sitemap|About US| Contact US: help@patsnap.com