Double-loss value network deep reinforcement learning KVFD model mechanical parameter global optimization method and system
A technology of reinforcement learning and mechanical parameters, applied in neural learning methods, biological neural network models, design optimization/simulation, etc., can solve problems such as difficulty in approaching global parameters, poor performance, and poor reliability
- Summary
- Abstract
- Description
- Claims
- Application Information
AI Technical Summary
Problems solved by technology
Method used
Image
Examples
specific Embodiment
[0065] In the embodiment of the present invention, the nano-indentation equation of the circular probe of the KVFD model (hereinafter referred to as the KVFD equation) is selected to implement the strategy of the present invention. The equation is divided into three loading protocols: Ramp-relaxation, Load-unload, and Ramp-creep (hereinafter referred to as relaxation, load-unload, and creep). The equation system is shown in Table 1.
[0066] Table 1. Nanoindentation equation of KVFD model under circular probe
[0067]
[0068] The mechanical parameters to be optimized are [E 0 , α, τ]. R is the probe radius, v is the indentation depth increasing speed during loading, k is the pressure increasing speed during loading, T r is the turning time, Γ( ) is the gamma function, is the complete beta function, is an incomplete beta function, is the Mittag-Leffler (M-L) function.
[0069] Construction of CF-DQN algorithm examples for three loading protocols for KVFD equations...
PUM
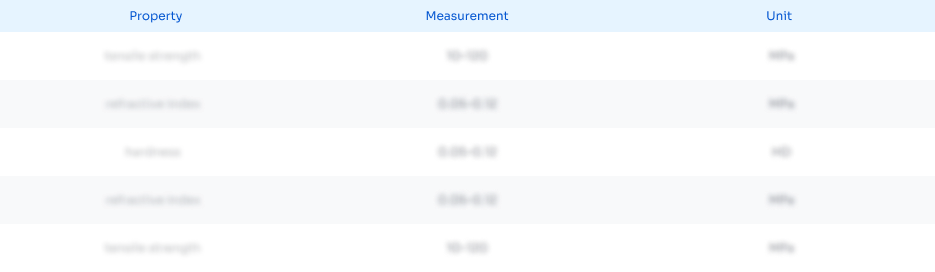
Abstract
Description
Claims
Application Information
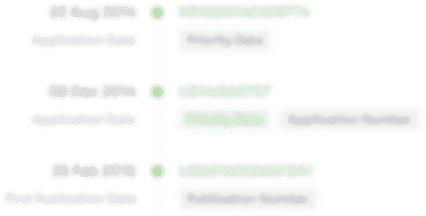
- R&D Engineer
- R&D Manager
- IP Professional
- Industry Leading Data Capabilities
- Powerful AI technology
- Patent DNA Extraction
Browse by: Latest US Patents, China's latest patents, Technical Efficacy Thesaurus, Application Domain, Technology Topic.
© 2024 PatSnap. All rights reserved.Legal|Privacy policy|Modern Slavery Act Transparency Statement|Sitemap