Method, system, storage medium and equipment for deep neural network countermeasure defense based on feature denoising
A deep neural network and equipment technology, applied in neural learning methods, biological neural network models, mechanical equipment, etc., can solve the problems of poor defense against samples and incomplete denoising, achieve good defense effects and ensure accurate classification The effect of improving the rate and improving the robustness of the confrontation
- Summary
- Abstract
- Description
- Claims
- Application Information
AI Technical Summary
Problems solved by technology
Method used
Image
Examples
specific Embodiment approach 1
[0057] 2a) Use ResNet34 as the main network architecture and benchmark comparison model.
[0062]
[0063] Among them, θ is the model weight parameter, x is the original clean sample, δ is the adversarial disturbance, and y is the standard of the original clean sample
[0064] 3b) The optimization algorithm used in network training is SGD with momentum, the initial learning rate is set to 0.1, and the
[0074] Figure 2 is a structural diagram of the feature denoising module, which is mainly composed of three parts: 1×1 convolution, residual connection and denoising operation. That
[0076] Figure 4 is a diagram of the FSDResNet34 network model structure, that is, two Figure 2 are embedded in the ResNet34 residual network.
[0079]
[0080]
[0081] where δ represents the standard deviation of the noise, and N represents the signal length or image size, since the
[0084]
[0090]
[0091]
[0095]z
[0099]
[0100]
[0102]
[0105]
[0108]
[0111] In view of the fact that the ...
specific Embodiment approach 2
[0124] Figure 20 is the adversarial sample (PGD) generated by the clean sample in ResNet34, and Figure 21 is the adversarial sample in
specific Embodiment approach 3
PUM
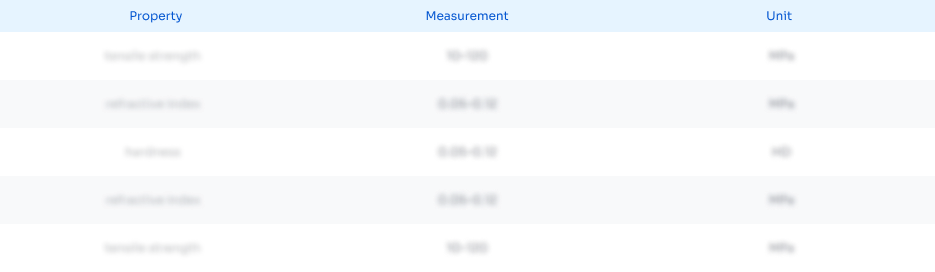
Abstract
Description
Claims
Application Information
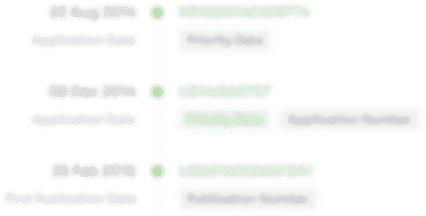
- R&D Engineer
- R&D Manager
- IP Professional
- Industry Leading Data Capabilities
- Powerful AI technology
- Patent DNA Extraction
Browse by: Latest US Patents, China's latest patents, Technical Efficacy Thesaurus, Application Domain, Technology Topic, Popular Technical Reports.
© 2024 PatSnap. All rights reserved.Legal|Privacy policy|Modern Slavery Act Transparency Statement|Sitemap|About US| Contact US: help@patsnap.com