A soft-threshold defense method for remote sensing image adversarial samples
A technology against samples and remote sensing images, applied in neural learning methods, instruments, biological neural network models, etc., can solve problems such as large amount of calculations, achieve simple and effective algorithms, and resist anti-deception effects
- Summary
- Abstract
- Description
- Claims
- Application Information
AI Technical Summary
Problems solved by technology
Method used
Image
Examples
Embodiment Construction
[0042] The present invention will be further described below in conjunction with the accompanying drawings, but the present invention is not limited in any way. Any transformation or replacement based on the teaching of the present invention belongs to the protection scope of the present invention.
[0043] According to the attack selectivity of RSI adversarial samples, the key to the soft threshold defense method is to correctly obtain the confidence threshold of each category. When the output confidence is higher than the threshold, it means that the input RSI is safe; while when the output confidence is lower than the threshold, the RSI may be an adversarial example, which is not safe.
[0044] like figure 1 As shown, a soft threshold defense method for remote sensing image adversarial samples disclosed in the present invention specifically includes the following steps:
[0045] S10: Save the correctly classified remote sensing image and the corresponding output confidence...
PUM
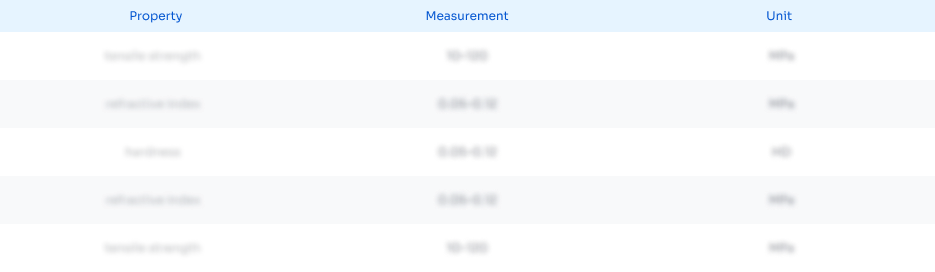
Abstract
Description
Claims
Application Information
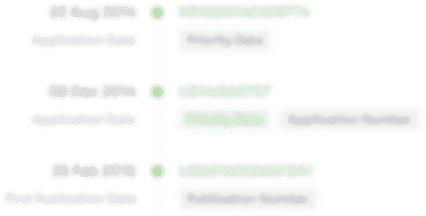
- R&D
- Intellectual Property
- Life Sciences
- Materials
- Tech Scout
- Unparalleled Data Quality
- Higher Quality Content
- 60% Fewer Hallucinations
Browse by: Latest US Patents, China's latest patents, Technical Efficacy Thesaurus, Application Domain, Technology Topic, Popular Technical Reports.
© 2025 PatSnap. All rights reserved.Legal|Privacy policy|Modern Slavery Act Transparency Statement|Sitemap|About US| Contact US: help@patsnap.com