Hyperspectral Image Classification Method Based on Spectral Spatial Attention Fusion and Deformable Convolutional Residual Networks
A hyperspectral image and spectral space technology, applied in the field of hyperspectral image classification, can solve the problems of insufficient spectral and spatial feature extraction, low classification accuracy of hyperspectral images, etc. The effect of accurate classification and enhanced generalization ability
- Summary
- Abstract
- Description
- Claims
- Application Information
AI Technical Summary
Problems solved by technology
Method used
Image
Examples
specific Embodiment approach 1
[0034] Specific implementation mode 1: In this implementation mode, the specific process of the hyperspectral image classification method based on spectral spatial attention fusion and deformable convolutional residual network is as follows:
[0035] Spectral information and spatial information are equally important for hyperspectral image classification. Many studies have shown that considering both feature extraction methods is much better than relying on only one of them. According to different stages of spectral feature fusion, these methods can be classified into three categories: preprocessing-based networks, ensemble networks, and post-processing-based networks.
[0036] Preprocessing based network
[0037] The classification process based on preprocessing usually includes two stages: 1) spectral-spatial feature extraction and fusion stage; 2) the extracted features are classified by different classifiers (such as SVM). The first stage is critical to determine the per...
specific Embodiment approach 2
[0054] Specific embodiment 2: The difference between this embodiment and specific embodiment 1 is that in the step 2, SSAF-DCR based on spectral spatial attention fusion and deformable convolution residual network includes:
[0055] The first input layer, the first unit, the second output layer, the sixth batch of normalization layer, the first hidden layer Dropout, the third input layer, the second unit, the thirteenth three-dimensional convolution, the thirteenth batch of normalization layer, second hidden layer Dropout, fifth input layer, third unit, global average pooling layer, fully connected layer;
[0056] The first unit includes: a first three-dimensional convolutional layer, a spectrally dense block, a fifth normalization layer, a fifth PReLU activation layer, a sixth three-dimensional convolutional layer, and a spectral attention block;
[0057] The spectrally dense block includes: the first batch of normalization layers, the first PReLU activation layer, the second...
specific Embodiment approach 3
[0064] Specific embodiment three: the difference between this embodiment and specific embodiment one or two is that the SSAF-DCR connection relationship based on spectral spatial attention fusion and deformable convolutional residual network is:
[0065] The output layer of the first input layer is connected to the input of the first three-dimensional convolutional layer in the first unit, and the output of the first three-dimensional convolutional layer is respectively used as the input of the first batch of normalization layers in the spectral dense block, and the second batch of The input of the normalization layer, the input of the third batch of normalization layer and the input of the fifth batch of normalization layer;
[0066] The output of the first batch of normalization layers is connected to the input of the first PReLU activation layer, the output of the first PReLU activation layer is connected to the input of the second three-dimensional convolutional layer, and ...
PUM
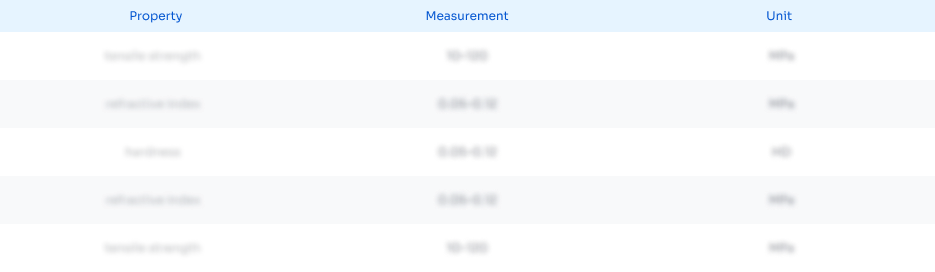
Abstract
Description
Claims
Application Information
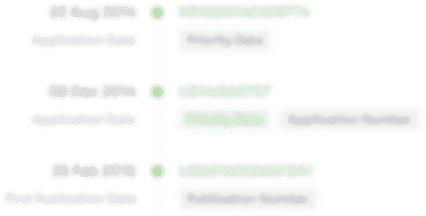
- R&D Engineer
- R&D Manager
- IP Professional
- Industry Leading Data Capabilities
- Powerful AI technology
- Patent DNA Extraction
Browse by: Latest US Patents, China's latest patents, Technical Efficacy Thesaurus, Application Domain, Technology Topic, Popular Technical Reports.
© 2024 PatSnap. All rights reserved.Legal|Privacy policy|Modern Slavery Act Transparency Statement|Sitemap|About US| Contact US: help@patsnap.com