Header column entity relationship matching method based on deep learning multi-head selection model
A technology of model selection and deep learning, applied in the direction of neural learning methods, biological neural network models, and other database retrievals, can solve problems such as ignoring the semantics of table headers and high startup costs of the scheme, and achieve the effect of low startup costs
- Summary
- Abstract
- Description
- Claims
- Application Information
AI Technical Summary
Problems solved by technology
Method used
Image
Examples
Embodiment
[0027] Example: such as Figure 1-2 As shown, the present invention is based on the table header column entity relationship matching method of the deep learning multi-head selection model, including several steps:
[0028] Step 1: Define data entity attribute categories for the data items in the table, such as time, name, company name, etc., and build a regular recognition method;
[0029] Step 2: Construct the artificial features of any combination of two columns in the table header. The construction method of the artificial features can be selected according to the needs of the actual scene;
[0030] Step 3: After passing the header character sequence and the data attribute sequence corresponding to the header through the respective embedding layers, the merged vector is used as the input of the next encoding layer;
[0031] Step 4: The coding layer adopts the bi-lstm model structure, and the output is the code of each position of the header sequence fused with the context ...
PUM
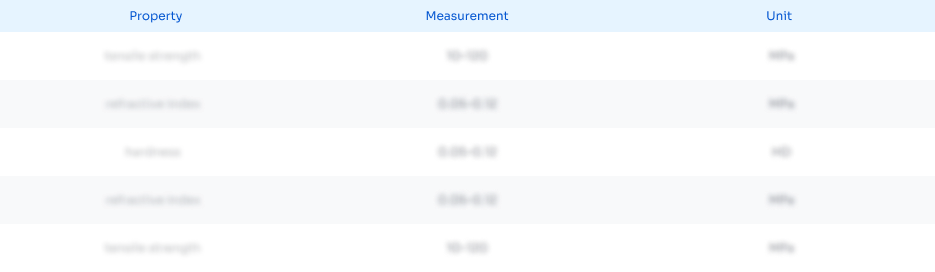
Abstract
Description
Claims
Application Information
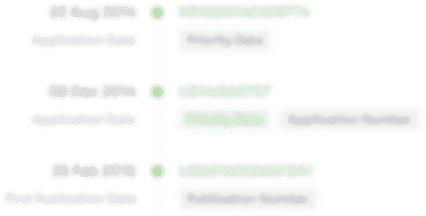
- R&D Engineer
- R&D Manager
- IP Professional
- Industry Leading Data Capabilities
- Powerful AI technology
- Patent DNA Extraction
Browse by: Latest US Patents, China's latest patents, Technical Efficacy Thesaurus, Application Domain, Technology Topic.
© 2024 PatSnap. All rights reserved.Legal|Privacy policy|Modern Slavery Act Transparency Statement|Sitemap