Hyperspectral remote sensing image classification method
A technology of hyperspectral remote sensing and image classification, applied in neural learning methods, instruments, biological neural network models, etc., can solve problems such as classification of difficult hyperspectral remote sensing images, improve classification performance and generalization ability, and expand data sets Effect
- Summary
- Abstract
- Description
- Claims
- Application Information
AI Technical Summary
Problems solved by technology
Method used
Image
Examples
Embodiment 1
[0154] In order to verify the influence of different parameter settings in the method proposed by the present invention on the classification accuracy, several factors that affect the classification effect of the model in the MSRA-G method will be analyzed, mainly including dimensionality reduction d, input sample window size w, and learning rate lr. The batch size is unified to 64, the iteration is 200 times, and the average value of the classification accuracy of 10 experiments is used as the experimental result.
[0155] Figure 5 It shows the impact of the classification method MSRA-G on the classification accuracy under different dimensions d in the present invention. from Figure 5 It can be observed that different dimensionality reduction d makes OA perform differently, basically showing a trend of first increasing and then decreasing. For the IP dataset, when d is 14, OA reaches the highest value, and then the OA value is basically stable. While the UP and SA data ...
Embodiment 2
[0158] Embodiment 2: In order to further verify the performance of the algorithm in this paper, this embodiment uses three data sets of IP, UP, and SV for verification, and randomly selects 5%, 1%, and 0.5% from each type of ground object as a training sample set, The rest are used as the test sample set. And use REF-SVM, 3D-CNN, MSDN, HybridSN, SSRN and R-HybridSN six hyperspectral image classification methods as the comparative experimental objects, the classification results take the mean of ten experimental results, and record the standard deviation, so as to verify MSRA - Classification performance of the G method.
[0159] Table 2 Comparison table of classification accuracy of different classification methods on IP dataset
[0160]
[0161]
[0162] right figure 2 The IP hyperspectral images shown are classified, and the MSRA-G classification method proposed in the present invention uses GANs to generate synthetic samples to achieve the purpose of expanding the ...
PUM
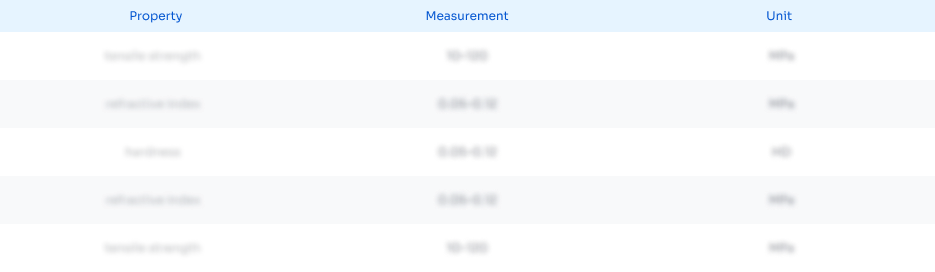
Abstract
Description
Claims
Application Information
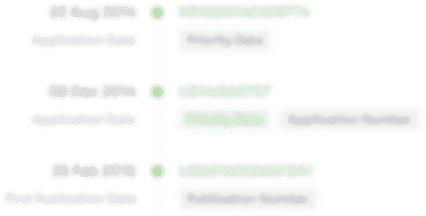
- Generate Ideas
- Intellectual Property
- Life Sciences
- Materials
- Tech Scout
- Unparalleled Data Quality
- Higher Quality Content
- 60% Fewer Hallucinations
Browse by: Latest US Patents, China's latest patents, Technical Efficacy Thesaurus, Application Domain, Technology Topic, Popular Technical Reports.
© 2025 PatSnap. All rights reserved.Legal|Privacy policy|Modern Slavery Act Transparency Statement|Sitemap|About US| Contact US: help@patsnap.com