Method for identifying individual subway bus taking and waiting behaviors by using mobile phone signaling data
A mobile phone signaling and subway technology, applied in the field of rail transit data analysis, can solve the problem of inability to refine the identification and analysis of individual subway travel behavior.
- Summary
- Abstract
- Description
- Claims
- Application Information
AI Technical Summary
Problems solved by technology
Method used
Image
Examples
Embodiment 1
[0047] At present, in the construction of the subway, the section of the subway network is not traceable, and the timeliness is low. The future subway lines will develop in a network trend, and more routes can be selected between the same OD. However, the granularity of traditional rail transit data analysis is only to the traffic starting and ending point OD, which is insufficient. To support the individual-level travel behavior analysis in the subway, it is impossible to analyze the real travel route selection and waiting behavior of the individual, and accurately identify the individual travel route, which is not conducive to the management and control of the subway operation and the accurate analysis of passenger flow. Based on this, On the basis of traditional rail transit data analysis, a method of using mobile phone signaling data to identify individual subway ride behavior is proposed in Embodiment 1. For the flow chart, see figure 1 , including the following steps:
...
Embodiment 2
[0069] Based on the MeanShift clustering performed according to the arrival time of all users passing through the research site described in Embodiment 1, in this embodiment, the specific process of identifying the group of fellow passengers on the line where the research site is located is described.
[0070] In the traditional K-Means algorithm, the final clustering effect is affected by the initial clustering center. The proposal of the K-Means++ algorithm provides a basis for selecting a better initial clustering center, but in the algorithm, the clustering The number of categories k still needs to be determined in advance. For data sets whose number of categories is unknown in advance, K-Means and K-Means++ will be difficult to accurately solve them.
[0071] In the application scenario of identification of fellow passengers in the subway, the number of subway operating shifts of the day cannot be known in advance. For this, this embodiment applies the improved clustering ...
Embodiment 3
[0081] In this example, based on the methods proposed in Example 1 and Example 2, on the basis of individual subway travel chain reconstruction and waiting behavior identification, the Guangzhou line network is analyzed from three levels: point, line, and plane. in,
[0082] Point: Calculate the number of waiting times at the starting station / the number of waiting times at the transfer station, analyze the proportion of waiting times at the station, and use it as the basis for judging the congestion situation at the station.
[0083] Line: identify the congestion section of the line network, collect statistics on the passenger flow of the section by time period, and extract the congestion peak period and the congestion section. Carry out congestion warning and look for alternative travel plans in congested sections.
[0084] Aspects: 1) Traceability of station control in congested sections, trace the origin of passenger stations in congested sections, and control passenger fl...
PUM
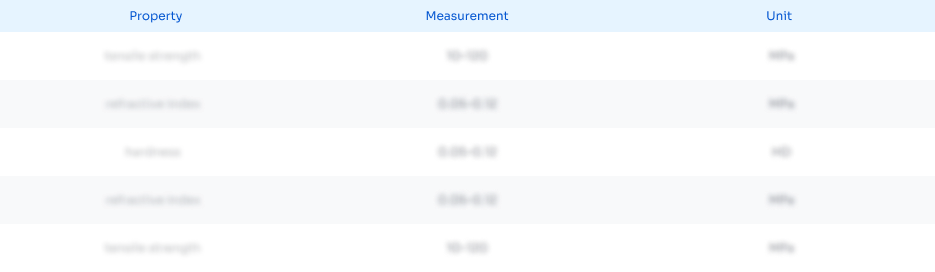
Abstract
Description
Claims
Application Information
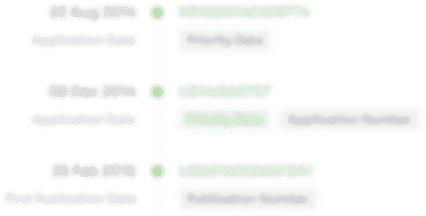
- R&D
- Intellectual Property
- Life Sciences
- Materials
- Tech Scout
- Unparalleled Data Quality
- Higher Quality Content
- 60% Fewer Hallucinations
Browse by: Latest US Patents, China's latest patents, Technical Efficacy Thesaurus, Application Domain, Technology Topic, Popular Technical Reports.
© 2025 PatSnap. All rights reserved.Legal|Privacy policy|Modern Slavery Act Transparency Statement|Sitemap|About US| Contact US: help@patsnap.com