Action prediction method based on human skeleton sequence
An action prediction and skeleton technology, applied in neural learning methods, instruments, biological neural network models, etc., can solve the problems of ignoring the potential of student models and failing to capture different importance, and achieve the effect of improving the classification of model predictions
- Summary
- Abstract
- Description
- Claims
- Application Information
AI Technical Summary
Problems solved by technology
Method used
Image
Examples
Embodiment Construction
[0050] In order to make the objectives, technical solutions and advantages of the present invention clearer, the present invention will be further described in detail below with reference to the embodiments. It should be understood that the specific embodiments described herein are only used to explain the present invention, but not to limit the present invention.
[0051] This embodiment provides an action prediction method based on human skeleton sequence, see figure 1 , the method includes the following steps:
[0052] S1: Use the graph convolutional network model to extract the spatiotemporal features of the human skeleton sequence data to be predicted, and input the spatiotemporal features into the trained multi-generation student model to obtain multiple classification results;
[0053] S2: Integrate multiple classification results to obtain the final prediction result.
[0054] Among them, the result fusion in step S2 can adopt the method of weighted fusion, which can...
PUM
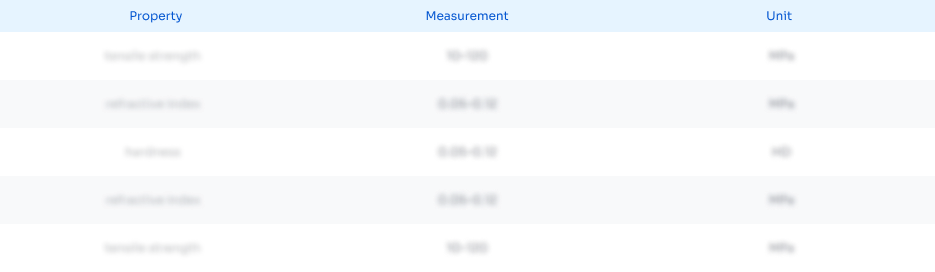
Abstract
Description
Claims
Application Information
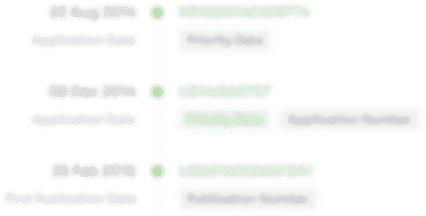
- R&D
- Intellectual Property
- Life Sciences
- Materials
- Tech Scout
- Unparalleled Data Quality
- Higher Quality Content
- 60% Fewer Hallucinations
Browse by: Latest US Patents, China's latest patents, Technical Efficacy Thesaurus, Application Domain, Technology Topic, Popular Technical Reports.
© 2025 PatSnap. All rights reserved.Legal|Privacy policy|Modern Slavery Act Transparency Statement|Sitemap|About US| Contact US: help@patsnap.com