A graph neural network representation learning-based structure graph alignment method and a multi-graph joint data mining method
A neural network and structure diagram technology, applied in the information field, can solve the problems of insufficient expression ability, lack of expression ability, simple modeling, etc., and achieve the effect of improving expression ability, good scalability, and stable training.
- Summary
- Abstract
- Description
- Claims
- Application Information
AI Technical Summary
Problems solved by technology
Method used
Image
Examples
Embodiment Construction
[0029] In order to make the above objects, features, and advantages of the present invention, the present invention will be further described in further detail by way of specific embodiments and the accompanying drawings.
[0030] The figure shows that learning is a method of excavating information, the goal is to learn the low-dimensional vector representation of the node in the figure, which can result in a simple operation for the target task. In the alignment, the target is to learn the low-dimensional vector representation of the node, and the similarity of the nodes between the two figures can be measured directly through the distance between the vectors. Compared to the similarities between the two figures, the learning map represents a lower complexity, more conducive to large-scale expansion, so this patent focuses on learning diagram representing vector to resolve the diagram alignment problem. Also because in real life, the complete attribute information on the graph is...
PUM
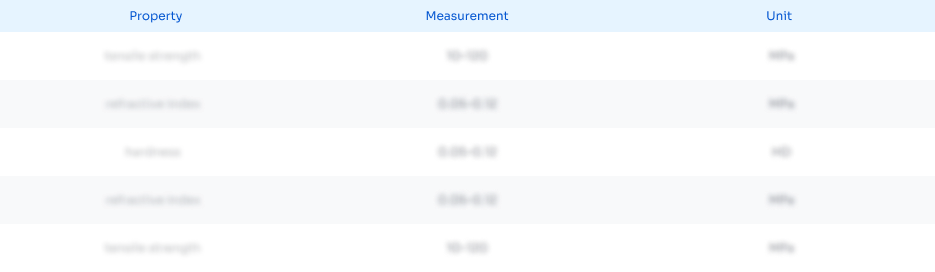
Abstract
Description
Claims
Application Information
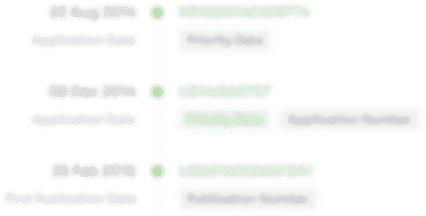
- R&D Engineer
- R&D Manager
- IP Professional
- Industry Leading Data Capabilities
- Powerful AI technology
- Patent DNA Extraction
Browse by: Latest US Patents, China's latest patents, Technical Efficacy Thesaurus, Application Domain, Technology Topic, Popular Technical Reports.
© 2024 PatSnap. All rights reserved.Legal|Privacy policy|Modern Slavery Act Transparency Statement|Sitemap|About US| Contact US: help@patsnap.com