Method, device and equipment for detecting anomaly of time series data and medium
A technology of time series and data anomalies, applied in the computer field, can solve problems such as dependence on the accuracy of budget algorithms, obvious dependence on human judgment, abnormal historical data/non-abnormal manual labeling, etc., and achieve the effect of accurately judging whether an abnormality occurs
- Summary
- Abstract
- Description
- Claims
- Application Information
AI Technical Summary
Problems solved by technology
Method used
Image
Examples
Embodiment
[0080] For a time series produced in the production server process, assuming that there are m sampling points in a time period, divide the time series into samples x of length m 1 ,x 2 ,…x n , where x i =(x i1 ,x i2 ,…x im ). where x 1 is the output at 1 o'clock, x 2 is the output at 2 o'clock, x 3 is the output at 3 o'clock, x 4 is the output at 4 o'clock, x m is the output at m o'clock. The specific implementation mode of the present invention can be divided into the following steps:
[0081] training phase, such as figure 2 shown:
[0082] 1. Determine the dimension of the abstract feature space, which can be set to any value, which is assumed to be 3 here.
[0083] 2. Determine the number of hidden layers and input and output dimensions in the encoder process. The number of layers and output dimensions can be set to any value. An initial value will be given at the beginning of training, and it will be dynamically adjusted according to the size of the loss fu...
PUM
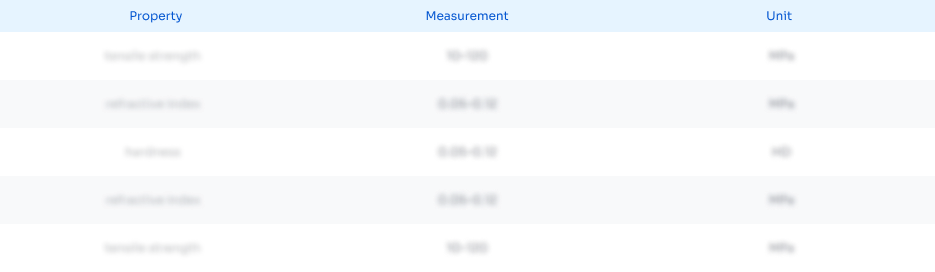
Abstract
Description
Claims
Application Information
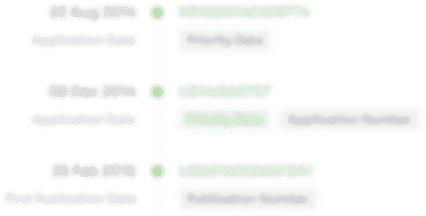
- R&D
- Intellectual Property
- Life Sciences
- Materials
- Tech Scout
- Unparalleled Data Quality
- Higher Quality Content
- 60% Fewer Hallucinations
Browse by: Latest US Patents, China's latest patents, Technical Efficacy Thesaurus, Application Domain, Technology Topic, Popular Technical Reports.
© 2025 PatSnap. All rights reserved.Legal|Privacy policy|Modern Slavery Act Transparency Statement|Sitemap|About US| Contact US: help@patsnap.com