Human face identification method based on manifold learning
A technology of face recognition and manifold learning, which is applied in the field of face recognition based on manifold learning, can solve the problems of not being able to discover the essential structure of high-dimensional face data, and not making good use of the local geometric information of the data, to achieve The effect of high face recognition rate
- Summary
- Abstract
- Description
- Claims
- Application Information
AI Technical Summary
Problems solved by technology
Method used
Image
Examples
Embodiment Construction
[0017] The embodiments of the present invention are described in detail below: the present embodiment is implemented under the premise of the technical solution of the present invention, and detailed implementation and specific operation process are provided, but the protection scope of the present invention is not limited to the following implementation example.
[0018] The embodiment adopts a public face database: ORL database. The ORL database contains 40 individuals with 10 images per person. The size of each image is 40×40. First stack the rows of the face image into a 1600-dimensional long vector, and then all the data form a 1600×400 matrix X=[x 1 ,L,x 400 ]. For each person, 5 samples are randomly selected for training and the rest are used for recognition. For each given number of training samples, 20 sets of random training-recognition sample sets are generated, and the average recognition rate is calculated on this basis. The present invention first finds 4 s...
PUM
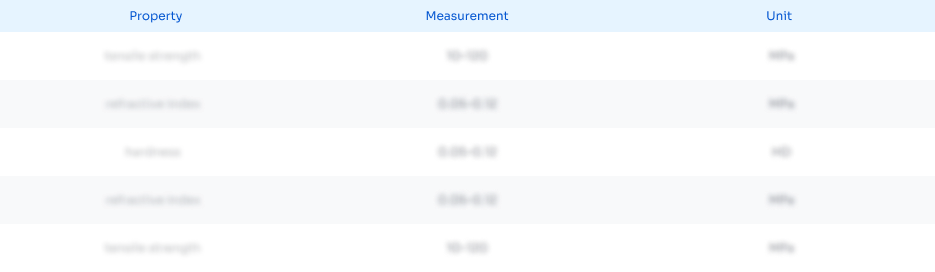
Abstract
Description
Claims
Application Information
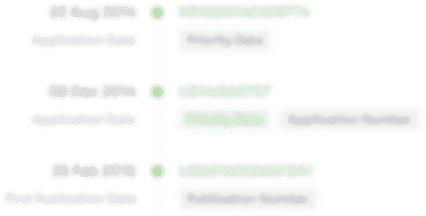
- R&D Engineer
- R&D Manager
- IP Professional
- Industry Leading Data Capabilities
- Powerful AI technology
- Patent DNA Extraction
Browse by: Latest US Patents, China's latest patents, Technical Efficacy Thesaurus, Application Domain, Technology Topic, Popular Technical Reports.
© 2024 PatSnap. All rights reserved.Legal|Privacy policy|Modern Slavery Act Transparency Statement|Sitemap|About US| Contact US: help@patsnap.com