Intrusion detection method based on observational learning
An observation learning and intrusion detection technology, applied in the field of pattern recognition and machine learning, can solve the problems of not effectively using unlabeled data, algorithm performance is not necessarily the best, and the process is tedious and boring, so as to improve the performance of the classifier and improve the overall performance. performance, confidence-enhancing effects
- Summary
- Abstract
- Description
- Claims
- Application Information
AI Technical Summary
Problems solved by technology
Method used
Image
Examples
Embodiment 1
[0041] The invention is an intrusion detection method based on observation learning, that is, an intrusion detection method based on SSELOLA (Semi-Supervised Ensemble Learning Based Observational Learning Algorithm).
[0042] The data used in the present invention is taken from an anomaly detection marked data set abbreviated KDDCUP99 provided by DARPA for the KDD (Knowledge Discovery and Data Mining) competition in 1999. The data set includes 5 million training sets and 3 million test sets. The data includes four types of attacks: Dos (denial of service attack), R2L (unauthorized remote access), U2R (unauthorized access to local super users) and Probe (scanning and detection), others are normal data. The present invention uses 13 of the 41 attributes. Since many of these user behavior features are redundant features, only 13 of them are selected to reflect the user behavior in order to simplify the problem.
[0043] The present invention selects all 52 pieces of data in the ...
Embodiment 2
[0062] The intrusion detection method based on observation and learning is the same as that in Example 1, taking 50% of the marked data in the training set of KDDCUP99 as an example, see figure 2 , the implementation process of the intrusion detection method based on SSELOLA is as follows:
[0063] Input: an unlabeled dataset x containing 147 data u , a labeled dataset X containing 146 data l , a test set T containing 2959 data.
[0064] Output: Classification error rate on the test set T.
[0065] (1) Select the backpropagation neural network algorithm with hidden units of 10, 20, 30, 40 and 50, and a total of five algorithms are denoted as L 1 , L 2 , L 3 , L 4 , L 5 .
[0066] (2) For the extracted unlabeled data set X containing 147 data u , a labeled dataset containing 146 data (initial training set) X l , a test set T containing 2959 data and five learning algorithms L 1 , L 2 , L 3 , L 4 , L 5 , combined with figure 2 to x l Algorithm L 1 ~ L 5 Perf...
Embodiment 3
[0075] The intrusion detection method based on observation and learning is the same as that in Embodiment 1-2, and the marked data in the training set of KDDCUP99 accounts for 20% as an example, see image 3 , the specific process is as follows:
[0076] Take out 59 data into the marked data set, take out 234 data into the unmarked data set, and put the remaining data into the test set. Five backpropagation neural network learning algorithms are used to train on the labeled data set, and five classifiers are obtained. For one classifier L among these five classifiers, observe and learn the output of the other four classifiers in each added unlabeled data set, and perform a majority vote based on these outputs to obtain a new labeled data set, Then add it to the training data set corresponding to the classifier, and remove this set of data in the corresponding unlabeled data set. Then use the new training data set to retrain the corresponding classifier, and then continue to ...
PUM
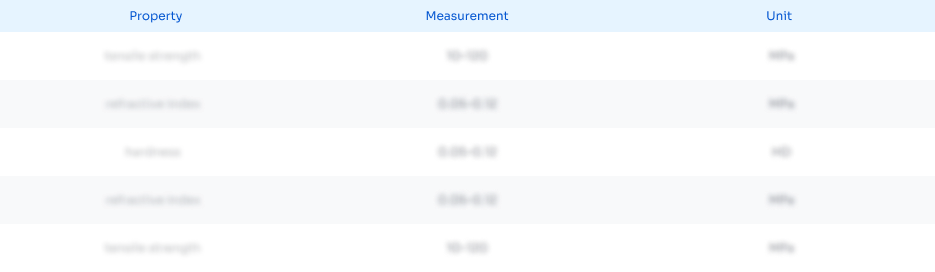
Abstract
Description
Claims
Application Information
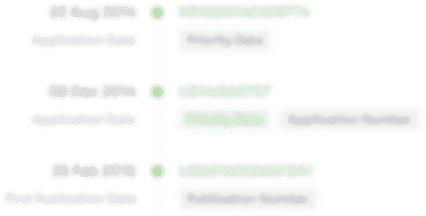
- R&D Engineer
- R&D Manager
- IP Professional
- Industry Leading Data Capabilities
- Powerful AI technology
- Patent DNA Extraction
Browse by: Latest US Patents, China's latest patents, Technical Efficacy Thesaurus, Application Domain, Technology Topic, Popular Technical Reports.
© 2024 PatSnap. All rights reserved.Legal|Privacy policy|Modern Slavery Act Transparency Statement|Sitemap|About US| Contact US: help@patsnap.com