Ultra-short-term prediction method of wind power based on self-learning composite data source
A composite data source and ultra-short-term forecasting technology, applied in machine learning, electrical digital data processing, special data processing applications, etc., can solve problems such as wind power, photovoltaic power generation output fluctuations, transmission network charging power fluctuations, etc., to improve prediction The effect of precision
- Summary
- Abstract
- Description
- Claims
- Application Information
AI Technical Summary
Problems solved by technology
Method used
Image
Examples
Embodiment Construction
[0055] The preferred embodiments of the present invention will be described below in conjunction with the accompanying drawings. It should be understood that the preferred embodiments described here are only used to illustrate and explain the present invention, and are not intended to limit the present invention.
[0056] A wind power ultra-short-term prediction method based on self-learning composite data sources, including input data to obtain autoregressive moving average model parameters;
[0057] Input the input data required for wind power prediction into the autoregressive moving average model determined according to the parameters of the autoregressive moving average model to obtain the prediction result;
[0058] Perform post-evaluation on the forecast results, that is, analyze the error between the predicted value and the measured value. If the forecast error is greater than the maximum allowable error, then re-establish the model order and model parameter estimation;...
PUM
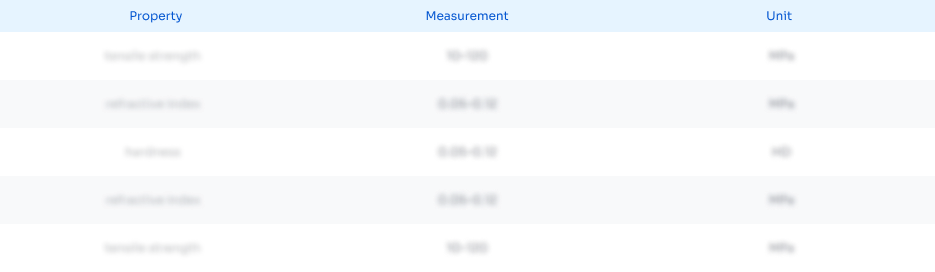
Abstract
Description
Claims
Application Information
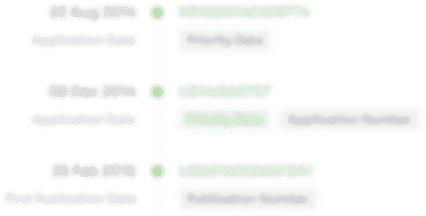
- R&D
- Intellectual Property
- Life Sciences
- Materials
- Tech Scout
- Unparalleled Data Quality
- Higher Quality Content
- 60% Fewer Hallucinations
Browse by: Latest US Patents, China's latest patents, Technical Efficacy Thesaurus, Application Domain, Technology Topic, Popular Technical Reports.
© 2025 PatSnap. All rights reserved.Legal|Privacy policy|Modern Slavery Act Transparency Statement|Sitemap|About US| Contact US: help@patsnap.com