Ultra-short-term power load prediction method and device based on machine learning
A technology of electric load and machine learning, which is applied in the direction of load forecasting, machine learning, and circuit devices in the AC network, can solve the problem of low forecasting accuracy, achieve the effects of ensuring forecasting efficiency, improving forecasting accuracy, and avoiding time-consuming effects
- Summary
- Abstract
- Description
- Claims
- Application Information
AI Technical Summary
Problems solved by technology
Method used
Image
Examples
Embodiment Construction
[0029] The present invention is further explained in detail below.
[0030] refer to figure 1 , an ultra-short-term power load forecasting method based on machine learning, the steps are as follows:
[0031] The first step is to normalize the original data;
[0032] Normalize the raw data using the peak method, the formula is as follows:
[0033]
[0034] Where: X i represents the original load, X max means X i the maximum value of Indicates the normalized value, and i represents the serial number.
[0035] The second step is to use the variational mode decomposition (VMD) method to process the load data, and decompose the highly volatile original load data into multiple relatively stable intrinsic mode function (IMF) components;
[0036] The parameters of variational mode decomposition (VMD) have a great influence on the decomposition effect, so the important parameters should be determined before decomposition. First, according to previous research results and pr...
PUM
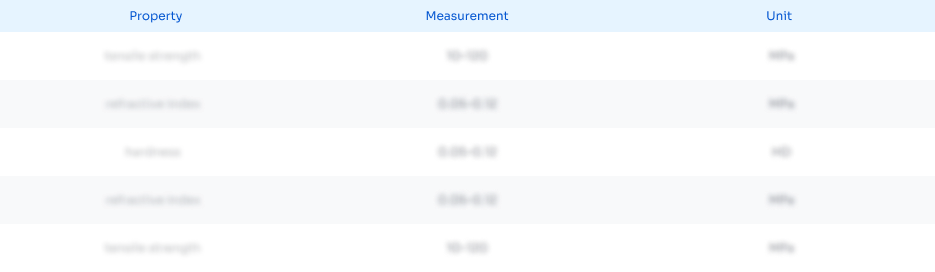
Abstract
Description
Claims
Application Information
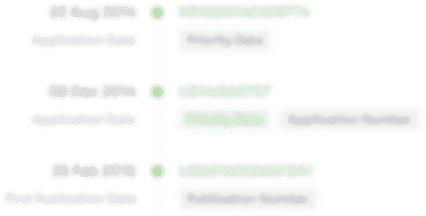
- R&D
- Intellectual Property
- Life Sciences
- Materials
- Tech Scout
- Unparalleled Data Quality
- Higher Quality Content
- 60% Fewer Hallucinations
Browse by: Latest US Patents, China's latest patents, Technical Efficacy Thesaurus, Application Domain, Technology Topic, Popular Technical Reports.
© 2025 PatSnap. All rights reserved.Legal|Privacy policy|Modern Slavery Act Transparency Statement|Sitemap|About US| Contact US: help@patsnap.com