Hyperspectral remote sensing data classification method based on ensemble learning
A technology of hyperspectral remote sensing and integrated learning, which is applied in the field of spectral data classification, can solve the problem of low data classification accuracy, and achieve the effect of improving classification effect, high classification accuracy, and high data classification accuracy
- Summary
- Abstract
- Description
- Claims
- Application Information
AI Technical Summary
Problems solved by technology
Method used
Image
Examples
specific Embodiment approach 1
[0030] Specific implementation mode one: the following combination figure 1 Describe this embodiment, the hyperspectral remote sensing data classification method based on integrated learning described in this embodiment, it comprises the following steps:
[0031] Step 1: Read the hyperspectral remote sensing data, use the principal component analysis method to calculate the eigenvalues and eigenvectors of the hyperspectral remote sensing data, and then obtain the spectral characteristics of the hyperspectral remote sensing data; then use the gray level co-occurrence matrix to extract the high Spatial features of spectral remote sensing data;
[0032] Step 2: Integrate spectral features and spatial features into a multi-feature set;
[0033] Step 3: Determine the labeled samples from the multi-feature set and select training samples and test samples;
[0034] Step 4: Based on the integrated learning method, design the Adaboost integrated classification framework with featur...
specific Embodiment approach 2
[0037] Specific implementation mode two: this implementation mode further explains implementation mode one, and the method for obtaining the spectral features of the hyperspectral remote sensing data described in this implementation mode is:
[0038] First, calculate the covariance matrix of the hyperspectral remote sensing data, and use the principal component analysis method to obtain the eigenvalues and eigenvectors of all the data according to the covariance matrix, and arrange the corresponding eigenvectors according to the order of the eigenvalues from large to small, and Use the eigenvector as the weighting coefficient to calculate and obtain B principal component components, B is a positive integer; select the first N principal component components as the spectral features of the hyperspectral remote sensing data, and N is the value after rounding B / 2;
[0039] The method for obtaining the spatial characteristics of hyperspectral remote sensing data is as follows: e...
specific Embodiment approach 3
[0044] Specific implementation mode three: this implementation mode further explains implementation mode two, and the method for obtaining the multi-feature set in step two described in this implementation mode is:
[0045] Randomly select D numbers from 1 to N, D<N, extract the corresponding one-dimensional spectral features and two-dimensional spatial feature data according to the D number numbers in the spectral features and spatial features, and form a multi-dimensional matrix representation Feature set; repeat this process F-1 times to obtain F multi-feature sets.
[0046] Integrate spectral and spatial features into a multi-feature set. Such as figure 1 As shown in , in order to facilitate the subsequent integrated classification, F multi-feature sets need to be formed. Multi-feature sets include spectral features and spatial features. D digit numbers are expressed as {d 1 , d 2 ,...,d D}.
PUM
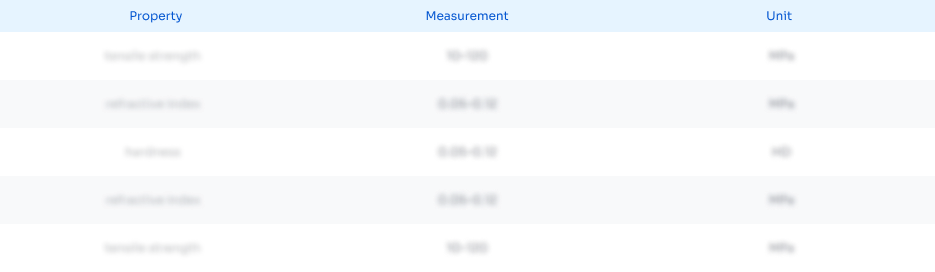
Abstract
Description
Claims
Application Information
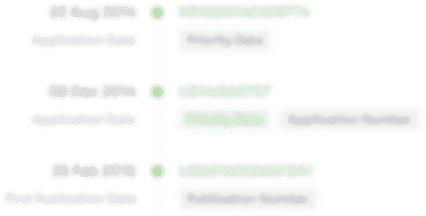
- R&D
- Intellectual Property
- Life Sciences
- Materials
- Tech Scout
- Unparalleled Data Quality
- Higher Quality Content
- 60% Fewer Hallucinations
Browse by: Latest US Patents, China's latest patents, Technical Efficacy Thesaurus, Application Domain, Technology Topic, Popular Technical Reports.
© 2025 PatSnap. All rights reserved.Legal|Privacy policy|Modern Slavery Act Transparency Statement|Sitemap|About US| Contact US: help@patsnap.com