Massive image classification method based on deep vector of locally aggregated descriptors (VLAD)
A technology of local features and classification methods, applied in the field of image processing and deep learning, to achieve the effect of improving accuracy
- Summary
- Abstract
- Description
- Claims
- Application Information
AI Technical Summary
Problems solved by technology
Method used
Image
Examples
Embodiment Construction
[0021] The massive image classification method based on deep local feature descriptors proposed by the present invention specifically includes the following steps:
[0022] Step 1: Set the entire deep learning model as the L-layer deep learning process; extract the SIFT feature of each training picture, and the SIFT feature set S of the entire training sample set is expressed as:
[0023] S=[s 1 ,...,S N ], S∈R D×N
[0024] Where N is the number of SIFT features in the SIFT feature set, D is the dimension of each SIFT feature; Kmeans clustering is performed on the SIFT feature set S to obtain the dictionary of the first layer of deep learning process D 1 ∈R D×K , Where K is the dictionary D 1 The number of cluster centers in, D is the dimension of the cluster centers;
[0025] Step 2: Define that each training picture is a set of T=M1×M2×M3 image blocks B=[B 1 ,..., B t ,..., B T ], that is, each training picture has M3 sub-regions, each sub-region is composed of M1×M2 image blocks;...
PUM
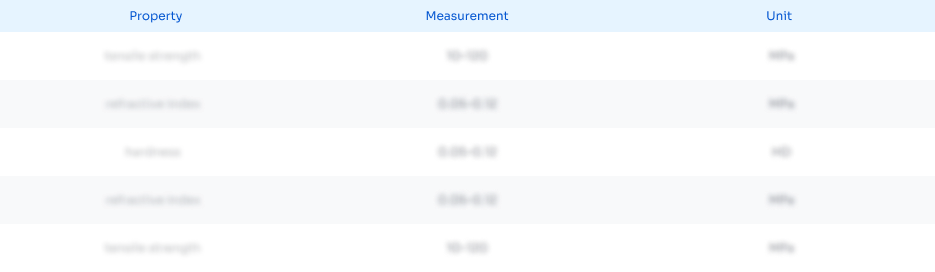
Abstract
Description
Claims
Application Information
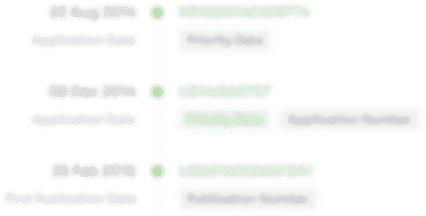
- R&D Engineer
- R&D Manager
- IP Professional
- Industry Leading Data Capabilities
- Powerful AI technology
- Patent DNA Extraction
Browse by: Latest US Patents, China's latest patents, Technical Efficacy Thesaurus, Application Domain, Technology Topic, Popular Technical Reports.
© 2024 PatSnap. All rights reserved.Legal|Privacy policy|Modern Slavery Act Transparency Statement|Sitemap|About US| Contact US: help@patsnap.com