Feature selection method based on Laplacian operator
A feature selection method and operator technology, applied in computing, computer parts, instruments, etc., can solve the problems of ignoring the interdependence between samples and samples, affecting the discriminability of selected features, and affecting the classification performance of classifiers.
- Summary
- Abstract
- Description
- Claims
- Application Information
AI Technical Summary
Problems solved by technology
Method used
Image
Examples
Embodiment Construction
[0037] Embodiments of the present invention are described in detail below, examples of which are shown in the drawings, wherein the same or similar reference numerals denote the same or similar elements or elements having the same or similar functions throughout. The embodiments described below by referring to the figures are exemplary only for explaining the present invention and should not be construed as limiting the present invention.
[0038] Below in conjunction with embodiment technical scheme of the present invention is described in further detail:
[0039] A specific embodiment of the present invention lists evaluating the effectiveness of the proposed method on 8 UCI data sets. Table 1 lists the characteristics of these datasets.
[0040] Table 1 Datasets used in the experiments
[0041]
[0042] We first compare Lasso's feature selection methods, and compare the classic ranking-based feature selection methods, including Laplacian Score (LS) and FisherScore (FS)...
PUM
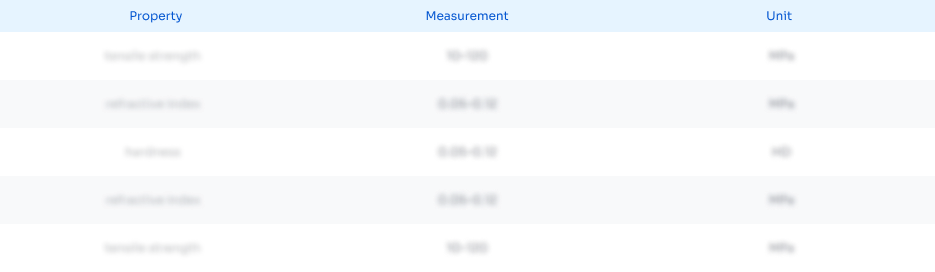
Abstract
Description
Claims
Application Information
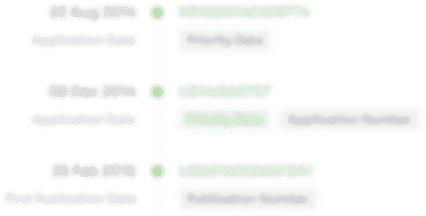
- R&D
- Intellectual Property
- Life Sciences
- Materials
- Tech Scout
- Unparalleled Data Quality
- Higher Quality Content
- 60% Fewer Hallucinations
Browse by: Latest US Patents, China's latest patents, Technical Efficacy Thesaurus, Application Domain, Technology Topic, Popular Technical Reports.
© 2025 PatSnap. All rights reserved.Legal|Privacy policy|Modern Slavery Act Transparency Statement|Sitemap|About US| Contact US: help@patsnap.com