Click and vision fusion based weak supervision bilinear deep learning method
A deep learning and weakly supervised technology, applied in the field of weakly supervised bilinear deep learning, can solve the problems of high labor cost and lack of prospects, and achieve the effect of improving the effect.
- Summary
- Abstract
- Description
- Claims
- Application Information
AI Technical Summary
Problems solved by technology
Method used
Image
Examples
Embodiment Construction
[0053] The present invention will be further described in detail below in conjunction with the accompanying drawings.
[0054] Such as figure 1 As shown, a weakly supervised bilinear deep learning method based on click and visual fusion, specifically includes the following steps:
[0055] Step (1) extracts the click feature corresponding to the image from the click data set and merges it according to semantic clustering, as follows:
[0056] 1-1. In order to meet the experimental needs, we separately extract all dog-related samples from the click data set Clickture provided by Microsoft to form a new data set Clickture-Dog. The data set has 344 pictures of dogs, and we filter the categories with less than 5 pictures, and finally get 283 sets of pictures. Then, the data set is split into training, validation, and testing in the form of 5:3:2. In order to improve the imbalance of the number of pictures in each class during training, we will select a class with more than 300 p...
PUM
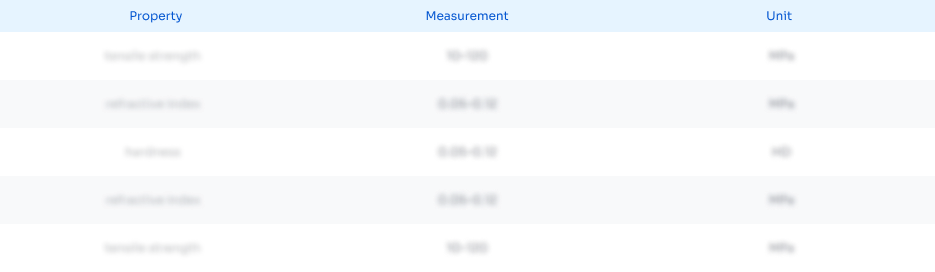
Abstract
Description
Claims
Application Information
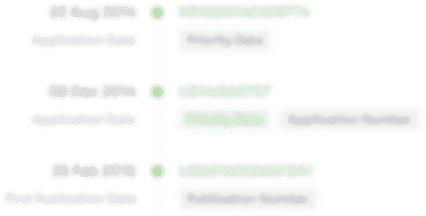
- R&D Engineer
- R&D Manager
- IP Professional
- Industry Leading Data Capabilities
- Powerful AI technology
- Patent DNA Extraction
Browse by: Latest US Patents, China's latest patents, Technical Efficacy Thesaurus, Application Domain, Technology Topic, Popular Technical Reports.
© 2024 PatSnap. All rights reserved.Legal|Privacy policy|Modern Slavery Act Transparency Statement|Sitemap|About US| Contact US: help@patsnap.com