Unsupervised Segmentation of Natural Images Based on Mean Shift and Fuzzy Clustering
A natural image and mean shift technology, applied in the field of image processing, can solve problems such as image over-segmentation or under-segmentation, errors, and complex natural image scenes, etc., to achieve the effect of improving suppression ability, improving consistency, and suppressing influence
- Summary
- Abstract
- Description
- Claims
- Application Information
AI Technical Summary
Problems solved by technology
Method used
Image
Examples
Embodiment Construction
[0038] refer to figure 1 , the implementation steps of the present invention are as follows:
[0039] Step 1: Normalize the image to be segmented.
[0040] Enter as figure 2 The natural image I shown in (a) t , i=1,2,...,n, n represents the number of images to be divided in the image data set; for image I iThe RGB value of the pixel is normalized so that the value of each color channel is in the range [0,1], figure 2 (a) The distribution of image pixels in the normalized RGB color space is as follows: figure 2 as shown in (b);
[0041] Step 2: Use the following smoothing formula to image I t To smooth:
[0042]
[0043] in, Indicates the k+1th iteration value of the central pixel of the i-th sliding window, and the end condition of the sliding window iteration is N i Represents the set of all pixels in the sliding window, s i Represents the spatial coordinates of the pixel at the center of the sliding window, s j Indicates the neighborhood pixel space coord...
PUM
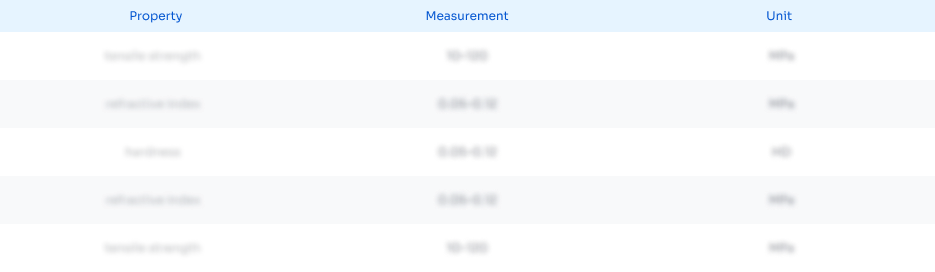
Abstract
Description
Claims
Application Information
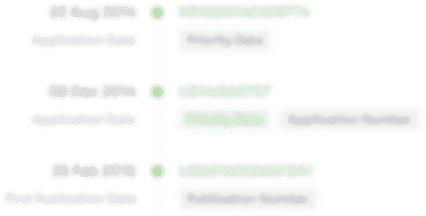
- R&D Engineer
- R&D Manager
- IP Professional
- Industry Leading Data Capabilities
- Powerful AI technology
- Patent DNA Extraction
Browse by: Latest US Patents, China's latest patents, Technical Efficacy Thesaurus, Application Domain, Technology Topic, Popular Technical Reports.
© 2024 PatSnap. All rights reserved.Legal|Privacy policy|Modern Slavery Act Transparency Statement|Sitemap|About US| Contact US: help@patsnap.com