Network model compression method and device based on multi-granularity pruning
A network model and multi-granularity technology, which is applied in the field of video surveillance, deep neural network, and image processing, can solve the problem of not being able to significantly reduce storage and computing resources, and achieve the effect of reducing computing consumption and compressing size
- Summary
- Abstract
- Description
- Claims
- Application Information
AI Technical Summary
Problems solved by technology
Method used
Image
Examples
Embodiment Construction
[0036] In order to enable your examiners to further understand the structure, features and other purposes of the present invention, the attached preferred embodiments are now described in detail as follows. The described preferred embodiments are only used to illustrate the technical solutions of the present invention, not to limit the present invention. invention.
[0037] The network model compression method based on multi-granularity pruning according to the present invention comprises the following one or two or three steps:
[0038] The step of pruning the granularity level of the input channel adopts the unimportant element pruning method to prune the unimportant elements in the granularity level of the input channel of the network model;
[0039] The pruning step of the granularity level of the convolution kernel adopts the unimportant element pruning method to prune the unimportant elements in the granularity level of the convolution kernel of the network model;
[00...
PUM
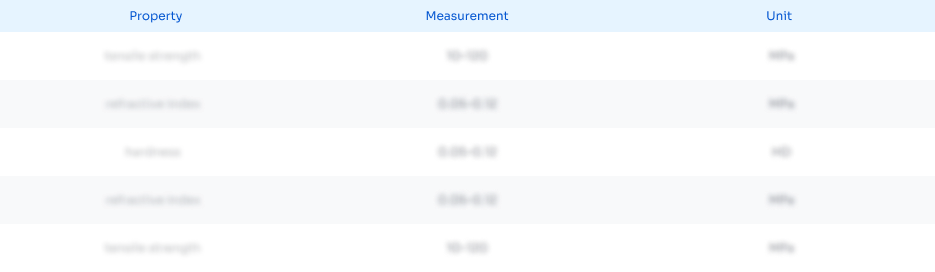
Abstract
Description
Claims
Application Information
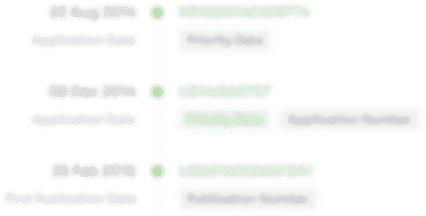
- R&D Engineer
- R&D Manager
- IP Professional
- Industry Leading Data Capabilities
- Powerful AI technology
- Patent DNA Extraction
Browse by: Latest US Patents, China's latest patents, Technical Efficacy Thesaurus, Application Domain, Technology Topic, Popular Technical Reports.
© 2024 PatSnap. All rights reserved.Legal|Privacy policy|Modern Slavery Act Transparency Statement|Sitemap|About US| Contact US: help@patsnap.com