A Depth Estimation Method for Monocular Image Based on Fully Convolutional Neural Network FCN
A convolutional neural network and image depth technology, which is applied in the field of monocular image depth estimation, can solve the problems of low resolution and insufficient precision of the resulting image, reduce the amount of parameters, optimize the structure, and improve the output image with low resolution. Effect
- Summary
- Abstract
- Description
- Claims
- Application Information
AI Technical Summary
Problems solved by technology
Method used
Image
Examples
Embodiment Construction
[0025] Embodiments of the present invention will be described below in conjunction with the accompanying drawings.
[0026] Such as figure 1 As shown, the present invention designs a monocular image depth estimation method based on a fully convolutional neural network FCN, and trains an end-to-end prediction and estimation system based on a fully convolutional neural network, eliminating the need for post-processing steps in traditional methods , which is simpler and more practical. This method specifically comprises the following steps:
[0027] Step 1. Obtain training image data.
[0028] Since the network layer is relatively deep and the number of parameters required for training is large, the amount of training data that needs to be prepared needs to meet a certain order of magnitude requirement. In the indoor scene, the NYU Depth V2 data set is used as a basis to generate the final required training data. Use the original image data of 249 scenes in the 464 indoor sce...
PUM
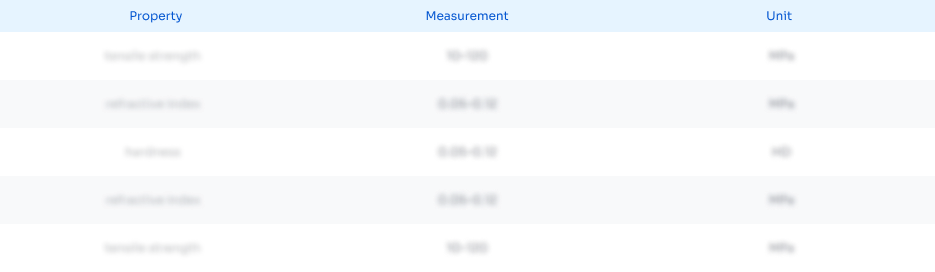
Abstract
Description
Claims
Application Information
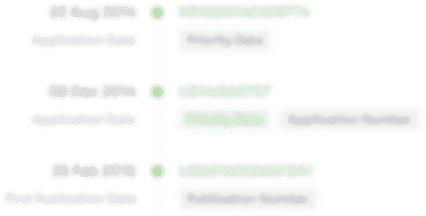
- R&D
- Intellectual Property
- Life Sciences
- Materials
- Tech Scout
- Unparalleled Data Quality
- Higher Quality Content
- 60% Fewer Hallucinations
Browse by: Latest US Patents, China's latest patents, Technical Efficacy Thesaurus, Application Domain, Technology Topic, Popular Technical Reports.
© 2025 PatSnap. All rights reserved.Legal|Privacy policy|Modern Slavery Act Transparency Statement|Sitemap|About US| Contact US: help@patsnap.com