Adversarial-based lightweight network semantic segmentation method
A semantic segmentation, lightweight technology, applied in neural learning methods, biological neural network models, image analysis and other directions, can solve problems such as the accuracy rate is only 58%, the real-time semantic segmentation accuracy has a large room for improvement, and the accuracy rate decreases.
- Summary
- Abstract
- Description
- Claims
- Application Information
AI Technical Summary
Problems solved by technology
Method used
Image
Examples
Embodiment Construction
[0047] In order to enable those skilled in the art to better understand and use the present invention, the technical solutions of the present invention will be further described below in conjunction with the accompanying drawings and specific implementation examples.
[0048] 1. Data preprocessing. Each image in the data set needs to have its corresponding labeled image, and the images are divided into three groups: training, verification and testing. These data set images are horizontally flipped, randomly cropped, and multi-scale transformed to obtain preprocessed images.
[0049] 2. The overall structure of the confrontation-based lightweight network semantic segmentation method proposed by the present invention is as follows: figure 1 As shown, it mainly includes two parts: (1) given the input image, the predicted label is generated by the lightweight segmentation network; (2) the discriminant network distinguishes the predicted label from the real label, and the probabil...
PUM
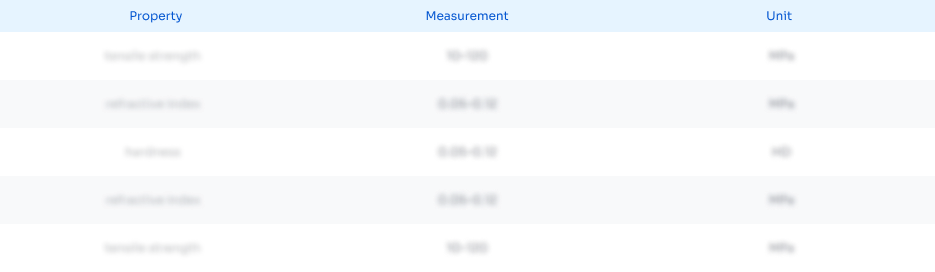
Abstract
Description
Claims
Application Information
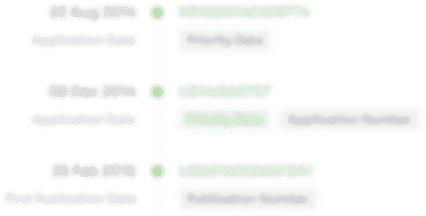
- R&D Engineer
- R&D Manager
- IP Professional
- Industry Leading Data Capabilities
- Powerful AI technology
- Patent DNA Extraction
Browse by: Latest US Patents, China's latest patents, Technical Efficacy Thesaurus, Application Domain, Technology Topic, Popular Technical Reports.
© 2024 PatSnap. All rights reserved.Legal|Privacy policy|Modern Slavery Act Transparency Statement|Sitemap|About US| Contact US: help@patsnap.com