Method for labeling and complementing gastric cancer pathological slice based on pseudo-label iterative annotation
A pathological slice and labeling technology, applied in image data processing, instrumentation, computing, etc., can solve the problem of incomplete labeling of gastric cancer pathological slices, achieve better prediction results, increase robustness, and improve quantity and quality.
- Summary
- Abstract
- Description
- Claims
- Application Information
AI Technical Summary
Problems solved by technology
Method used
Image
Examples
Embodiment Construction
[0027] In order to further understand the present invention, a method for annotating and completing gastric cancer pathological slices based on pseudo-label iterative labeling provided by the present invention will be described in detail below in conjunction with specific embodiments, but the present invention is not limited thereto. Non-essential improvements and adjustments made under the guiding ideology still belong to the protection scope of the present invention.
[0028] Such as figure 1 As shown, the specific label iterative labeling process of the present invention is:
[0029] 1) Generate pseudo-label samples
[0030] Firstly, obtain negative sample slices (denoted as N) and incompletely labeled positive sample slices (denoted as P) in the data set (gastric cancer pathological slices), segment the marked lesions in P, and record the corresponding s position.
[0031] The lesion parts obtained above are spliced to the corresponding positions in N to generate a ga...
PUM
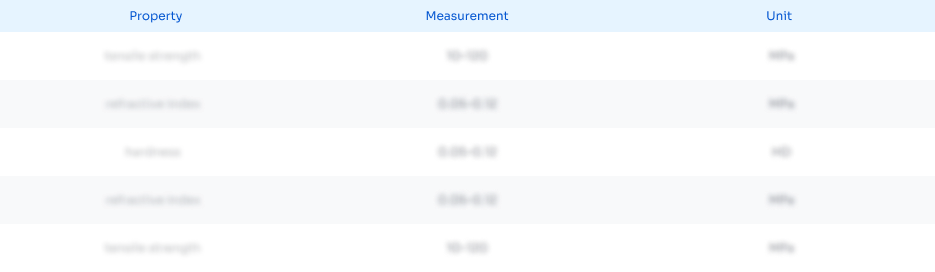
Abstract
Description
Claims
Application Information
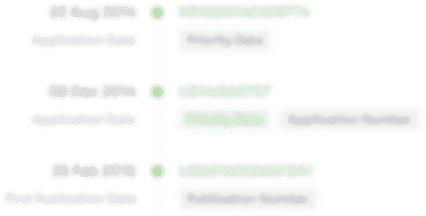
- R&D Engineer
- R&D Manager
- IP Professional
- Industry Leading Data Capabilities
- Powerful AI technology
- Patent DNA Extraction
Browse by: Latest US Patents, China's latest patents, Technical Efficacy Thesaurus, Application Domain, Technology Topic, Popular Technical Reports.
© 2024 PatSnap. All rights reserved.Legal|Privacy policy|Modern Slavery Act Transparency Statement|Sitemap|About US| Contact US: help@patsnap.com