A meta-learning algorithm based on stepwise gradient correction of a meta-learner
A gradient correction and meta-learning technology, applied in the field of deep neural networks, can solve problems such as difficulty in reproduction, construction of complex meta-learners, and incomprehension of meta-learning algorithms
- Summary
- Abstract
- Description
- Claims
- Application Information
AI Technical Summary
Problems solved by technology
Method used
Image
Examples
Embodiment Construction
[0063] The implementation of the present invention will be described in detail below in conjunction with the drawings and examples.
[0064] Such as figure 1 As shown, the present invention is a meta-learning algorithm based on the gradual gradient correction of the meta-learner, which is used to train the classifier on the training data with noisy labels. Great for real data scenarios with noisy markers. First, obtain a training dataset with noisy labels and a small amount of clean and unbiased metadata set; compared to the classifier (student network) built on the training dataset, build a meta-learner (teacher network) on the metadata set ; jointly update the student network and teacher network parameters using stochastic gradient descent. That is, the student network parameter gradient update function is obtained through the student network gradient descent format; it is fed back to the teacher network, and the teacher network parameter update is obtained by using the me...
PUM
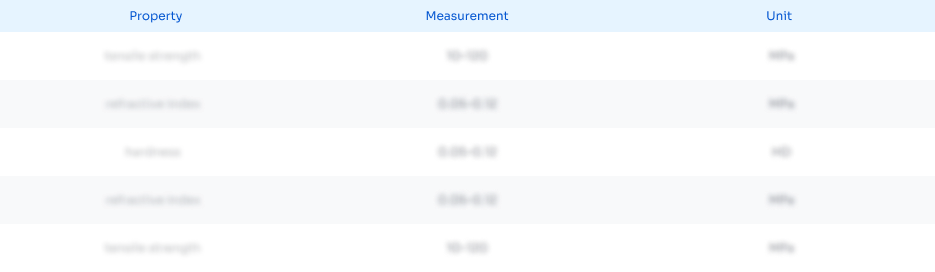
Abstract
Description
Claims
Application Information
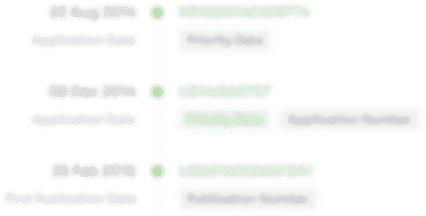
- R&D Engineer
- R&D Manager
- IP Professional
- Industry Leading Data Capabilities
- Powerful AI technology
- Patent DNA Extraction
Browse by: Latest US Patents, China's latest patents, Technical Efficacy Thesaurus, Application Domain, Technology Topic, Popular Technical Reports.
© 2024 PatSnap. All rights reserved.Legal|Privacy policy|Modern Slavery Act Transparency Statement|Sitemap|About US| Contact US: help@patsnap.com