A Driving Risk Prediction Method Based on Time-varying State Transition Probability Markov Chain
A Markov chain and transition probability technology, applied in data processing applications, instruments, calculations, etc., can solve the problems of a single early warning parameter, the inability to fully reflect the inherent evolution law of the driving state, the accuracy of the adverse driving risk model and the prediction accuracy.
- Summary
- Abstract
- Description
- Claims
- Application Information
AI Technical Summary
Problems solved by technology
Method used
Image
Examples
Embodiment Construction
[0039] The present invention will be further described below with reference to the accompanying drawings, but the protection scope of the present invention is not limited thereto.
[0040] like figure 1 As shown, a driving risk prediction method based on the time-varying state transition probability Markov chain, including the steps:
[0041] Step 1: Offline driving risk prediction model training: On the basis of natural driving database accident and nearby accident samples, select the time window feature parameters based on vehicle driving characteristics, and divide the real-time driving risk status by clustering the feature parameters and use them as Markov chain can list the states; based on the time window characteristic parameters and driver, road, and environmental variable parameters under different driving risk states, establish a multinomial logistic model of driving risk state transition under different driving risk states;
[0042] The offline driving risk predict...
PUM
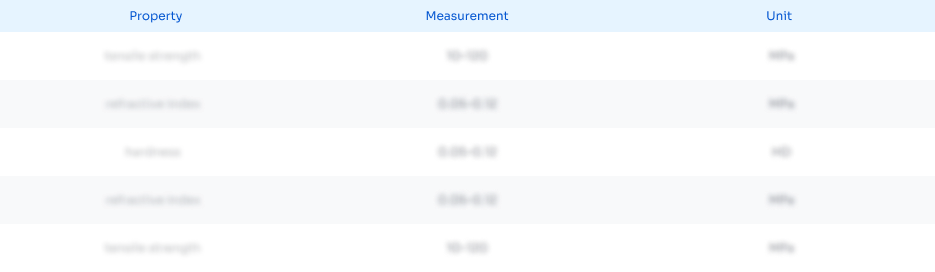
Abstract
Description
Claims
Application Information
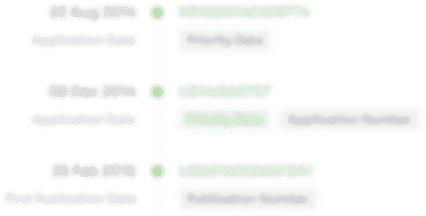
- R&D Engineer
- R&D Manager
- IP Professional
- Industry Leading Data Capabilities
- Powerful AI technology
- Patent DNA Extraction
Browse by: Latest US Patents, China's latest patents, Technical Efficacy Thesaurus, Application Domain, Technology Topic, Popular Technical Reports.
© 2024 PatSnap. All rights reserved.Legal|Privacy policy|Modern Slavery Act Transparency Statement|Sitemap|About US| Contact US: help@patsnap.com